Lending Club Loan Analysis
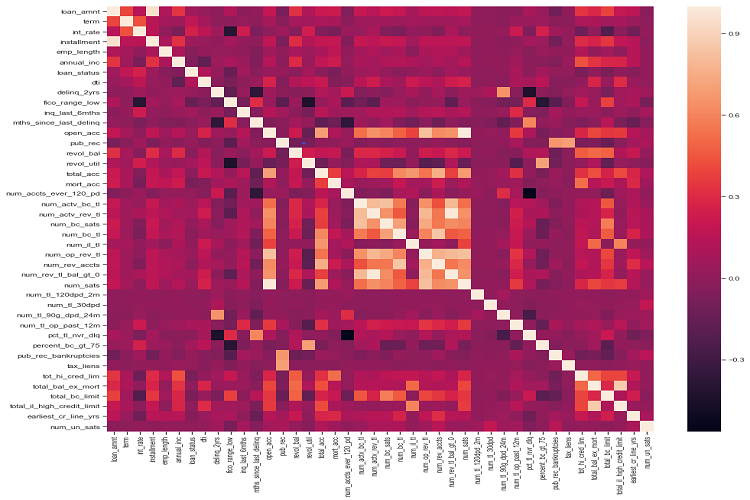
Data from Lending Club would be having sampling bias, as this dataset is of people already granted loan, so they have been already gone through one level of risk screening
Identify loans that may default and its reason
1
2
3
4
5
6
7
8
9
10
11
12
13
14
15
16
17
18
19
20
21
22
23
24
25
26
27
28
29
30
31
32
33
34
35
36
37
38
39
40
41
42
43
44
45
46
47
48
49
%%time
# Import required libraries
%matplotlib inline
import pandas as pd
import numpy as np
import matplotlib.pyplot as plt
import seaborn as sns
import re
import os
pd.options.display.max_columns = 200
pd.options.display.max_rows = 200
from sklearn.preprocessing import OrdinalEncoder, StandardScaler
import datetime
print(datetime.datetime.now())
from sklearn.model_selection import train_test_split, GridSearchCV
from sklearn.linear_model import LogisticRegression, SGDClassifier
from sklearn.svm import SVC
from sklearn.naive_bayes import GaussianNB
from sklearn.model_selection import cross_val_score, KFold
from sklearn.neighbors import KNeighborsClassifier
from sklearn.calibration import CalibratedClassifierCV
from sklearn.feature_selection import SelectFromModel
from sklearn.metrics import plot_roc_curve, classification_report
import featuretools as ft
from sklearn.externals import joblib
import xgboost as xgb
import lightgbm as lgb
import pickle
from sklearn.metrics import precision_score, recall_score, roc_auc_score, confusion_matrix, roc_curve, precision_recall_curve
import tensorflow as tf
from tensorflow import keras
from tensorflow.keras import layers
from imblearn.over_sampling import RandomOverSampler, SMOTE
from imblearn.under_sampling import RandomUnderSampler
import shap
shap.initjs()
1
2020-02-26 05:35:42.481520
1
Wall time: 17 ms
1
2
3
4
5
6
7
8
9
10
11
12
13
14
15
16
17
18
19
20
21
22
23
24
25
26
27
28
29
30
31
32
33
34
35
36
37
38
39
40
41
42
43
44
45
46
47
48
49
50
51
52
53
54
55
# Clean up data
good = 'Fully Paid'
bad = ['Charged Off', 'Default']
ignore = ['Current', 'Late (31-120 days)', 'In Grace Period', 'Late (16-30 days)']
# filter out columns that have over 85% of NaN
#df_2014 = pd.read_csv('../data/2014.csv')
#cols_to_keep=df_2014.columns[df_2014.isnull().sum()/df_2014.shape[0] < 0.85].tolist()
cols_to_keep=['id', 'loan_amnt', 'funded_amnt', 'funded_amnt_inv', 'term', 'int_rate', 'installment', 'grade', 'sub_grade', 'emp_title',
'emp_length', 'home_ownership', 'annual_inc', 'verification_status', 'issue_d', 'loan_status', 'pymnt_plan', 'url', 'purpose',
'title', 'zip_code', 'addr_state', 'dti', 'delinq_2yrs', 'earliest_cr_line', 'fico_range_low', 'fico_range_high', 'inq_last_6mths',
'mths_since_last_delinq', 'mths_since_last_record', 'open_acc', 'pub_rec', 'revol_bal', 'revol_util', 'total_acc',
'initial_list_status', 'out_prncp', 'out_prncp_inv', 'total_pymnt', 'total_pymnt_inv', 'total_rec_prncp', 'total_rec_int',
'total_rec_late_fee', 'recoveries', 'collection_recovery_fee', 'last_pymnt_d', 'last_pymnt_amnt', 'last_credit_pull_d',
'last_fico_range_high', 'last_fico_range_low', 'collections_12_mths_ex_med', 'mths_since_last_major_derog',
'policy_code', 'application_type', 'acc_now_delinq', 'tot_coll_amt', 'tot_cur_bal', 'total_rev_hi_lim', 'acc_open_past_24mths',
'avg_cur_bal', 'bc_open_to_buy', 'bc_util', 'chargeoff_within_12_mths', 'delinq_amnt', 'mo_sin_old_il_acct', 'mo_sin_old_rev_tl_op',
'mo_sin_rcnt_rev_tl_op', 'mo_sin_rcnt_tl', 'mort_acc', 'mths_since_recent_bc', 'mths_since_recent_bc_dlq', 'mths_since_recent_inq',
'mths_since_recent_revol_delinq', 'num_accts_ever_120_pd', 'num_actv_bc_tl', 'num_actv_rev_tl', 'num_bc_sats', 'num_bc_tl','num_il_tl',
'num_op_rev_tl', 'num_rev_accts', 'num_rev_tl_bal_gt_0', 'num_sats', 'num_tl_120dpd_2m', 'num_tl_30dpd', 'num_tl_90g_dpd_24m',
'num_tl_op_past_12m', 'pct_tl_nvr_dlq', 'percent_bc_gt_75', 'pub_rec_bankruptcies', 'tax_liens', 'tot_hi_cred_lim', 'total_bal_ex_mort',
'total_bc_limit', 'total_il_high_credit_limit', 'hardship_flag', 'disbursement_method', 'debt_settlement_flag']
# List of columns to drop that are not getting any value such as ID, URL or one that are duplicate
cols_to_drop = ['id', 'emp_title', 'pymnt_plan', 'url', 'fico_range_high','title', 'zip_code', 'mths_since_last_record', 'out_prncp_inv', 'total_pymnt',
'total_pymnt_inv', 'total_rec_prncp', 'total_rec_int', 'total_rec_late_fee', 'recoveries', 'collection_recovery_fee',
'last_pymnt_d', 'last_pymnt_amnt', 'last_credit_pull_d', 'last_fico_range_high', 'last_fico_range_low', 'out_prncp',
'collections_12_mths_ex_med', 'mths_since_last_major_derog', 'policy_code', 'application_type', 'acc_now_delinq',
'tot_coll_amt', 'tot_cur_bal', 'total_rev_hi_lim', 'acc_open_past_24mths', 'avg_cur_bal', 'bc_open_to_buy', 'bc_util',
'chargeoff_within_12_mths', 'delinq_amnt', 'mo_sin_old_il_acct', 'mo_sin_old_rev_tl_op', 'mo_sin_rcnt_rev_tl_op',
'mo_sin_rcnt_tl', 'mths_since_recent_bc', 'mths_since_recent_bc_dlq', 'mths_since_recent_inq',
'mths_since_recent_revol_delinq', 'hardship_flag', 'disbursement_method', 'debt_settlement_flag' ,'funded_amnt','funded_amnt_inv', 'initial_list_status']
def data_cleanup(df):
df = df[cols_to_keep]
df = df.loc[~df.loan_status.isin(ignore),]
df = df.drop(columns=cols_to_drop)
df.loan_status = df.loan_status.replace(bad,1)
df.loan_status = df.loan_status.replace(good,0)
df.mths_since_last_delinq= df.mths_since_last_delinq.fillna(999)
df.term = df.term.apply(lambda x: re.search(r'\d\d', x).group()).astype(np.uint8)
df.int_rate = df.int_rate.str.replace('%','').astype(np.float32)
df.revol_util = df.revol_util.str.replace('%','').astype(np.float32)
df.emp_length = df.emp_length.fillna(0).replace('[a-zA-Z+]*','',regex=True).replace('< 1',0,regex=True).astype(np.uint8)
df.num_tl_120dpd_2m = df.num_tl_120dpd_2m.fillna(0)
df.percent_bc_gt_75 = df.percent_bc_gt_75.fillna(0)
df.revol_util = df.revol_util.fillna(0)
# Get number of year of credit history at time of loan issue date
df['earliest_cr_line_yrs'] = df.earliest_cr_line.apply(lambda x: 100-int(x[-2:]) if int(x[-2:]) > 18 else int(x[-2:])*-1 ) + df.issue_d.apply(lambda x: x[-2:]).astype(np.uint8)
# Add a column for accounts in not satisfactory state
df['num_un_sats']=df.open_acc - df.num_sats
df.issue_d=pd.to_datetime(df.issue_d, format='%b-%y')
df = df.drop(columns='earliest_cr_line')
return df
1
2
3
4
5
6
7
8
9
10
11
12
13
14
%%time
# Loop through each data file and clean it up
data_files = ['../data/raw/{}'.format(i) for i in os.listdir('..\\data\\raw') if '201' in i]
df_all = pd.DataFrame()
for f in data_files:
print("Processing: {}".format(f))
temp_df = pd.read_csv(f)
temp_df = data_cleanup(temp_df)
df_all = pd.concat([df_all,temp_df])
print("Processesed. Shape of df_all: {}".format(df_all.shape))
df_all = df_all.reset_index(drop=True)
df_all.fillna(df_all.median(), inplace=True)
#df_all.to_csv('../data/processed/clean_data.csv', index=False)
1
2
3
4
5
6
7
8
9
10
11
12
13
14
15
16
17
18
19
20
21
22
23
24
25
26
27
28
29
30
31
Processing: ../data/raw/2012-13.csv
Columns (49,129,130,131,134,135,136,139) have mixed types.Specify dtype option on import or set low_memory=False.
Processesed. Shape of df_all: (181326, 49)
Processing: ../data/raw/2014.csv
Columns (19) have mixed types.Specify dtype option on import or set low_memory=False.
Processesed. Shape of df_all: (392092, 49)
Processing: ../data/raw/2015.csv
Columns (19,59) have mixed types.Specify dtype option on import or set low_memory=False.
Processesed. Shape of df_all: (637749, 49)
Processing: ../data/raw/2016.csv
Processesed. Shape of df_all: (795091, 49)
Processing: ../data/raw/2017.csv
Columns (118,129,130,131,134,135,136,139,145,146,147) have mixed types.Specify dtype option on import or set low_memory=False.
Processesed. Shape of df_all: (841160, 49)
Wall time: 33.8 s
1
df_all.loan_status.value_counts()[1]/(df_all.loan_status.value_counts()[0]+df_all.loan_status.value_counts()[1])
1
0.20993152313471872
1
df_all.head()
loan_amnt | term | int_rate | installment | grade | sub_grade | emp_length | home_ownership | annual_inc | verification_status | issue_d | loan_status | purpose | addr_state | dti | delinq_2yrs | fico_range_low | inq_last_6mths | mths_since_last_delinq | open_acc | pub_rec | revol_bal | revol_util | total_acc | mort_acc | num_accts_ever_120_pd | num_actv_bc_tl | num_actv_rev_tl | num_bc_sats | num_bc_tl | num_il_tl | num_op_rev_tl | num_rev_accts | num_rev_tl_bal_gt_0 | num_sats | num_tl_120dpd_2m | num_tl_30dpd | num_tl_90g_dpd_24m | num_tl_op_past_12m | pct_tl_nvr_dlq | percent_bc_gt_75 | pub_rec_bankruptcies | tax_liens | tot_hi_cred_lim | total_bal_ex_mort | total_bc_limit | total_il_high_credit_limit | earliest_cr_line_yrs | num_un_sats | |
---|---|---|---|---|---|---|---|---|---|---|---|---|---|---|---|---|---|---|---|---|---|---|---|---|---|---|---|---|---|---|---|---|---|---|---|---|---|---|---|---|---|---|---|---|---|---|---|---|---|
0 | 14000 | 36 | 12.85 | 470.71 | B | B4 | 4 | RENT | 88000.0 | Not Verified | 2013-12-01 | 0 | debt_consolidation | NC | 10.02 | 1 | 670 | 0.0 | 16.0 | 6 | 1 | 3686 | 81.900002 | 14 | 0.0 | 0.0 | 3.0 | 4.0 | 3.0 | 9.0 | 3.0 | 4.0 | 10.0 | 4.0 | 6.0 | 0.0 | 0.0 | 0.0 | 0.0 | 78.6 | 100.0 | 1 | 0 | 31840.0 | 17672.0 | 3900.0 | 27340.0 | 25 | 0.0 |
1 | 15000 | 36 | 14.47 | 516.10 | C | C2 | 10 | RENT | 98000.0 | Not Verified | 2013-12-01 | 0 | debt_consolidation | NY | 6.15 | 0 | 715 | 2.0 | 999.0 | 16 | 0 | 5749 | 22.299999 | 16 | 0.0 | 0.0 | 8.0 | 8.0 | 13.0 | 13.0 | 1.0 | 15.0 | 15.0 | 8.0 | 16.0 | 0.0 | 0.0 | 0.0 | 2.0 | 100.0 | 7.7 | 0 | 0 | 33300.0 | 13038.0 | 20800.0 | 7500.0 | 21 | 0.0 |
2 | 15000 | 36 | 8.90 | 476.30 | A | A5 | 2 | MORTGAGE | 63000.0 | Not Verified | 2013-12-01 | 0 | debt_consolidation | FL | 16.51 | 0 | 670 | 0.0 | 34.0 | 8 | 0 | 11431 | 74.199997 | 29 | 4.0 | 3.0 | 3.0 | 4.0 | 3.0 | 10.0 | 8.0 | 6.0 | 17.0 | 4.0 | 8.0 | 0.0 | 0.0 | 0.0 | 0.0 | 89.3 | 66.7 | 0 | 0 | 288195.0 | 39448.0 | 14200.0 | 33895.0 | 15 | 0.0 |
3 | 10000 | 36 | 9.67 | 321.13 | B | B1 | 7 | MORTGAGE | 102000.0 | Not Verified | 2013-12-01 | 0 | debt_consolidation | MA | 15.55 | 2 | 670 | 0.0 | 11.0 | 9 | 0 | 9912 | 44.400002 | 22 | 0.0 | 1.0 | 3.0 | 4.0 | 3.0 | 6.0 | 9.0 | 6.0 | 13.0 | 4.0 | 9.0 | 0.0 | 0.0 | 0.0 | 1.0 | 77.3 | 66.7 | 0 | 0 | 58486.0 | 39143.0 | 9200.0 | 36186.0 | 24 | 0.0 |
4 | 20800 | 36 | 13.53 | 706.16 | B | B5 | 10 | RENT | 81500.0 | Verified | 2013-12-01 | 0 | debt_consolidation | NY | 16.73 | 0 | 685 | 2.0 | 64.0 | 29 | 0 | 23473 | 54.500000 | 41 | 0.0 | 1.0 | 8.0 | 24.0 | 11.0 | 17.0 | 1.0 | 29.0 | 40.0 | 24.0 | 29.0 | 0.0 | 0.0 | 0.0 | 3.0 | 90.2 | 50.0 | 0 | 0 | 43100.0 | 23473.0 | 15000.0 | 0.0 | 15 | 0.0 |
1
2
3
4
5
6
7
8
9
10
11
12
13
14
15
16
17
18
19
20
21
22
23
24
25
26
27
28
29
30
31
32
33
34
35
36
37
38
39
40
41
42
43
44
#fig, ax = plt.subplots(2,2, figsize=(20,20))
#sns.distplot(np.log(df_all.annual_inc))
plt.figure(figsize=(20,12))
plt.subplot(2,3,1)
plt.hist(df_all.annual_inc[df_all.loan_status == 0], log=True)
plt.hist(df_all.annual_inc[df_all.loan_status == 1], log=True)
plt.xlabel("Annual Income (in logs)")
plt.title("Historgram of Annual Income (in logs)")
plt.subplot(2,3,2)
plt.hist(df_all.loan_amnt[df_all.loan_status == 0])
plt.hist(df_all.loan_amnt[df_all.loan_status == 1])
plt.xlabel("Loan Amount")
plt.title("Historgram of Loan Amount")
plt.subplot(2,3,3)
plt.hist(df_all.int_rate[df_all.loan_status == 0])
plt.hist(df_all.int_rate[df_all.loan_status == 1])
plt.xlabel("Interest Rate Charged")
plt.title("Interest Rate Charged")
plt.subplot(2,3,4)
plt.hist(df_all.installment[df_all.loan_status == 0])
plt.hist(df_all.installment[df_all.loan_status == 1])
plt.xlabel("Loan installment")
plt.title("Loan installment")
plt.subplot(2,3,5)
plt.hist(df_all.fico_range_low[df_all.loan_status == 0])
plt.hist(df_all.fico_range_low[df_all.loan_status == 1])
plt.xlabel("FICO Low")
plt.title("FICO Low")
plt.subplot(2,3,6)
plt.hist(df_all.revol_util[df_all.loan_status == 0])
plt.hist(df_all.revol_util[df_all.loan_status == 1])
plt.xlabel("Revolving Utilization")
plt.title("Revolving Utilization")
plt.show()
1
2
3
df_all.boxplot('loan_amnt', 'loan_status', figsize=(10,6))#, showfliers=False)
_ = plt.xlabel("Loan Staus 0=Good, 1=Defaulted")
_ = plt.ylabel("Loan Amount")
1
2
plt.figure(figsize=(16,8))
sns.boxplot(y='loan_amnt',x='grade', hue='loan_status',data=df_all.sort_values(by='grade'))
1
<matplotlib.axes._subplots.AxesSubplot at 0x2bf90fcd898>
1
2
3
_ = df_all.boxplot('annual_inc', 'loan_status', figsize=(10,6), showfliers=False)
_ = plt.xlabel("Loan Staus 0=Good, 1=Defaulted")
_ = plt.ylabel("Annual Income")
1
2
3
4
5
plt.figure(figsize=(12,6))
sns.set(style="ticks", palette="pastel")
sns.boxplot(x='grade', y='annual_inc',hue='loan_status', palette=['r','b'], data=df_all.sort_values(by='grade'), showfliers=False)
sns.despine(offset=10, trim=True)
1
_ = df_all.boxplot('revol_util', 'loan_status', figsize=(10,6), showfliers=False)
1
2
plt.figure(figsize=(12,6))
_ = sns.distplot(df_all.loan_amnt)
1
_=df_all.grade.value_counts().sort_index().plot.bar(figsize=(10,6))
1
2
3
4
plt.figure(figsize=(12,6))
sns.countplot(x='purpose', hue='loan_status', data=df_all)
plt.xticks(rotation=30)
1
2
(array([ 0, 1, 2, 3, 4, 5, 6, 7, 8, 9, 10, 11, 12, 13]),
<a list of 14 Text xticklabel objects>)
1
2
plt.figure(figsize=(12,6))
sns.countplot( x='grade', hue='loan_status', data=df_all.sort_values(by='grade'))
1
<matplotlib.axes._subplots.AxesSubplot at 0x2bf8e8b8748>
1
2
plt.figure(figsize=(12,8))
sns.barplot(x='grade',y='loan_status',data=df_all.sort_values(by='grade'))
1
<matplotlib.axes._subplots.AxesSubplot at 0x17bc119a630>
1
sns.catplot(x='grade', y='loan_amnt', hue='loan_status', data=df_all.sort_values(by='grade'), kind='bar')
1
<seaborn.axisgrid.FacetGrid at 0x17bc0058278>
1
sns.barplot(x='grade', y='loan_amnt', hue='loan_status', data=df_all.sort_values(by='grade'))
1
<matplotlib.axes._subplots.AxesSubplot at 0x17bb4c0d630>
1
corr=df_all.corr()
1
2
plt.figure(figsize=(16,16))
sns.heatmap(corr)
1
<matplotlib.axes._subplots.AxesSubplot at 0x17bb53c3cc0>
1
2
3
#highly co-relagted pairs
# loan_amnt - installment
# num_sats - open_acc
1
2
3
pd.concat([df_all[['num_sats', 'open_acc', 'loan_status']], df_all.num_sats - df_all.open_acc], axis=1)
#df_all.num_sats - df_all.open_acc
num_sats | open_acc | loan_status | 0 | |
---|---|---|---|---|
0 | 6.0 | 6 | 0 | 0.0 |
1 | 16.0 | 16 | 0 | 0.0 |
2 | 8.0 | 8 | 0 | 0.0 |
3 | 9.0 | 9 | 0 | 0.0 |
4 | 29.0 | 29 | 0 | 0.0 |
5 | 14.0 | 14 | 0 | 0.0 |
6 | 12.0 | 12 | 0 | 0.0 |
7 | 5.0 | 5 | 0 | 0.0 |
8 | 7.0 | 7 | 0 | 0.0 |
9 | 4.0 | 4 | 0 | 0.0 |
10 | 9.0 | 9 | 0 | 0.0 |
11 | 17.0 | 17 | 0 | 0.0 |
12 | 9.0 | 9 | 0 | 0.0 |
13 | 3.0 | 3 | 0 | 0.0 |
14 | 15.0 | 15 | 0 | 0.0 |
15 | 9.0 | 9 | 1 | 0.0 |
16 | 12.0 | 12 | 0 | 0.0 |
17 | 14.0 | 14 | 0 | 0.0 |
18 | 10.0 | 10 | 1 | 0.0 |
19 | 15.0 | 15 | 0 | 0.0 |
20 | 12.0 | 12 | 0 | 0.0 |
21 | 16.0 | 16 | 0 | 0.0 |
22 | 14.0 | 14 | 0 | 0.0 |
23 | 10.0 | 10 | 0 | 0.0 |
24 | 14.0 | 14 | 1 | 0.0 |
25 | 8.0 | 8 | 0 | 0.0 |
26 | 9.0 | 9 | 0 | 0.0 |
27 | 7.0 | 7 | 0 | 0.0 |
28 | 10.0 | 10 | 0 | 0.0 |
29 | 5.0 | 5 | 0 | 0.0 |
30 | 11.0 | 11 | 0 | 0.0 |
31 | 10.0 | 11 | 0 | -1.0 |
32 | 16.0 | 16 | 0 | 0.0 |
33 | 13.0 | 13 | 1 | 0.0 |
34 | 13.0 | 14 | 0 | -1.0 |
35 | 10.0 | 10 | 0 | 0.0 |
36 | 17.0 | 17 | 0 | 0.0 |
37 | 11.0 | 11 | 0 | 0.0 |
38 | 7.0 | 7 | 1 | 0.0 |
39 | 9.0 | 9 | 0 | 0.0 |
40 | 12.0 | 12 | 0 | 0.0 |
41 | 12.0 | 12 | 0 | 0.0 |
42 | 12.0 | 12 | 0 | 0.0 |
43 | 10.0 | 10 | 0 | 0.0 |
44 | 13.0 | 13 | 0 | 0.0 |
45 | 5.0 | 5 | 0 | 0.0 |
46 | 20.0 | 20 | 0 | 0.0 |
47 | 10.0 | 10 | 0 | 0.0 |
48 | 13.0 | 13 | 0 | 0.0 |
49 | 12.0 | 12 | 0 | 0.0 |
50 | 9.0 | 9 | 0 | 0.0 |
51 | 21.0 | 21 | 0 | 0.0 |
52 | 8.0 | 8 | 0 | 0.0 |
53 | 14.0 | 14 | 0 | 0.0 |
54 | 18.0 | 18 | 0 | 0.0 |
55 | 12.0 | 12 | 1 | 0.0 |
56 | 17.0 | 17 | 0 | 0.0 |
57 | 13.0 | 13 | 0 | 0.0 |
58 | 12.0 | 12 | 0 | 0.0 |
59 | 10.0 | 10 | 0 | 0.0 |
60 | 8.0 | 8 | 0 | 0.0 |
61 | 12.0 | 12 | 1 | 0.0 |
62 | 13.0 | 13 | 0 | 0.0 |
63 | 20.0 | 20 | 0 | 0.0 |
64 | 19.0 | 19 | 1 | 0.0 |
65 | 14.0 | 14 | 0 | 0.0 |
66 | 14.0 | 14 | 0 | 0.0 |
67 | 25.0 | 25 | 0 | 0.0 |
68 | 9.0 | 9 | 0 | 0.0 |
69 | 15.0 | 15 | 0 | 0.0 |
70 | 25.0 | 25 | 0 | 0.0 |
71 | 15.0 | 15 | 0 | 0.0 |
72 | 15.0 | 15 | 0 | 0.0 |
73 | 9.0 | 9 | 0 | 0.0 |
74 | 19.0 | 19 | 1 | 0.0 |
75 | 11.0 | 11 | 0 | 0.0 |
76 | 4.0 | 4 | 1 | 0.0 |
77 | 8.0 | 8 | 0 | 0.0 |
78 | 13.0 | 13 | 0 | 0.0 |
79 | 30.0 | 30 | 0 | 0.0 |
80 | 9.0 | 9 | 0 | 0.0 |
81 | 11.0 | 11 | 0 | 0.0 |
82 | 19.0 | 19 | 0 | 0.0 |
83 | 12.0 | 12 | 1 | 0.0 |
84 | 10.0 | 10 | 0 | 0.0 |
85 | 13.0 | 13 | 0 | 0.0 |
86 | 5.0 | 6 | 0 | -1.0 |
87 | 11.0 | 11 | 0 | 0.0 |
88 | 10.0 | 10 | 0 | 0.0 |
89 | 14.0 | 14 | 0 | 0.0 |
90 | 17.0 | 17 | 0 | 0.0 |
91 | 17.0 | 17 | 0 | 0.0 |
92 | 13.0 | 13 | 0 | 0.0 |
93 | 7.0 | 7 | 0 | 0.0 |
94 | 8.0 | 8 | 0 | 0.0 |
95 | 10.0 | 11 | 0 | -1.0 |
96 | 18.0 | 18 | 0 | 0.0 |
97 | 5.0 | 5 | 0 | 0.0 |
98 | 27.0 | 27 | 0 | 0.0 |
99 | 5.0 | 5 | 0 | 0.0 |
... | ... | ... | ... | ... |
841060 | 12.0 | 12 | 0 | 0.0 |
841061 | 12.0 | 12 | 0 | 0.0 |
841062 | 17.0 | 17 | 0 | 0.0 |
841063 | 7.0 | 7 | 0 | 0.0 |
841064 | 10.0 | 10 | 0 | 0.0 |
841065 | 16.0 | 16 | 0 | 0.0 |
841066 | 13.0 | 13 | 0 | 0.0 |
841067 | 12.0 | 12 | 0 | 0.0 |
841068 | 11.0 | 11 | 0 | 0.0 |
841069 | 16.0 | 16 | 0 | 0.0 |
841070 | 5.0 | 5 | 0 | 0.0 |
841071 | 9.0 | 9 | 0 | 0.0 |
841072 | 16.0 | 16 | 0 | 0.0 |
841073 | 6.0 | 6 | 0 | 0.0 |
841074 | 17.0 | 17 | 0 | 0.0 |
841075 | 11.0 | 11 | 0 | 0.0 |
841076 | 10.0 | 10 | 0 | 0.0 |
841077 | 13.0 | 13 | 0 | 0.0 |
841078 | 7.0 | 7 | 0 | 0.0 |
841079 | 17.0 | 17 | 0 | 0.0 |
841080 | 6.0 | 6 | 0 | 0.0 |
841081 | 10.0 | 10 | 0 | 0.0 |
841082 | 18.0 | 18 | 0 | 0.0 |
841083 | 11.0 | 11 | 0 | 0.0 |
841084 | 9.0 | 9 | 0 | 0.0 |
841085 | 13.0 | 13 | 0 | 0.0 |
841086 | 10.0 | 10 | 0 | 0.0 |
841087 | 12.0 | 12 | 0 | 0.0 |
841088 | 5.0 | 5 | 0 | 0.0 |
841089 | 14.0 | 14 | 0 | 0.0 |
841090 | 18.0 | 18 | 0 | 0.0 |
841091 | 4.0 | 4 | 0 | 0.0 |
841092 | 8.0 | 8 | 0 | 0.0 |
841093 | 7.0 | 7 | 0 | 0.0 |
841094 | 10.0 | 10 | 0 | 0.0 |
841095 | 13.0 | 13 | 0 | 0.0 |
841096 | 8.0 | 9 | 0 | -1.0 |
841097 | 12.0 | 12 | 0 | 0.0 |
841098 | 7.0 | 7 | 0 | 0.0 |
841099 | 2.0 | 2 | 0 | 0.0 |
841100 | 10.0 | 10 | 0 | 0.0 |
841101 | 14.0 | 14 | 0 | 0.0 |
841102 | 10.0 | 10 | 0 | 0.0 |
841103 | 23.0 | 23 | 0 | 0.0 |
841104 | 7.0 | 7 | 0 | 0.0 |
841105 | 10.0 | 10 | 0 | 0.0 |
841106 | 10.0 | 10 | 0 | 0.0 |
841107 | 5.0 | 5 | 0 | 0.0 |
841108 | 10.0 | 10 | 0 | 0.0 |
841109 | 15.0 | 15 | 0 | 0.0 |
841110 | 18.0 | 18 | 0 | 0.0 |
841111 | 19.0 | 19 | 0 | 0.0 |
841112 | 12.0 | 12 | 0 | 0.0 |
841113 | 14.0 | 14 | 0 | 0.0 |
841114 | 5.0 | 5 | 0 | 0.0 |
841115 | 9.0 | 9 | 0 | 0.0 |
841116 | 9.0 | 9 | 0 | 0.0 |
841117 | 11.0 | 11 | 0 | 0.0 |
841118 | 16.0 | 16 | 0 | 0.0 |
841119 | 9.0 | 9 | 0 | 0.0 |
841120 | 11.0 | 11 | 0 | 0.0 |
841121 | 3.0 | 3 | 0 | 0.0 |
841122 | 7.0 | 7 | 0 | 0.0 |
841123 | 12.0 | 12 | 0 | 0.0 |
841124 | 13.0 | 13 | 0 | 0.0 |
841125 | 11.0 | 11 | 0 | 0.0 |
841126 | 9.0 | 9 | 0 | 0.0 |
841127 | 24.0 | 24 | 0 | 0.0 |
841128 | 6.0 | 6 | 0 | 0.0 |
841129 | 14.0 | 14 | 0 | 0.0 |
841130 | 18.0 | 18 | 0 | 0.0 |
841131 | 24.0 | 24 | 0 | 0.0 |
841132 | 10.0 | 10 | 0 | 0.0 |
841133 | 6.0 | 6 | 0 | 0.0 |
841134 | 13.0 | 13 | 0 | 0.0 |
841135 | 5.0 | 5 | 0 | 0.0 |
841136 | 21.0 | 21 | 0 | 0.0 |
841137 | 8.0 | 8 | 0 | 0.0 |
841138 | 13.0 | 13 | 0 | 0.0 |
841139 | 16.0 | 16 | 0 | 0.0 |
841140 | 12.0 | 12 | 0 | 0.0 |
841141 | 12.0 | 12 | 0 | 0.0 |
841142 | 15.0 | 15 | 0 | 0.0 |
841143 | 14.0 | 14 | 0 | 0.0 |
841144 | 13.0 | 13 | 0 | 0.0 |
841145 | 8.0 | 8 | 0 | 0.0 |
841146 | 10.0 | 10 | 0 | 0.0 |
841147 | 13.0 | 13 | 0 | 0.0 |
841148 | 14.0 | 14 | 0 | 0.0 |
841149 | 12.0 | 12 | 0 | 0.0 |
841150 | 16.0 | 16 | 0 | 0.0 |
841151 | 14.0 | 14 | 0 | 0.0 |
841152 | 12.0 | 12 | 0 | 0.0 |
841153 | 3.0 | 3 | 0 | 0.0 |
841154 | 12.0 | 12 | 0 | 0.0 |
841155 | 10.0 | 10 | 0 | 0.0 |
841156 | 15.0 | 15 | 0 | 0.0 |
841157 | 20.0 | 20 | 0 | 0.0 |
841158 | 17.0 | 17 | 0 | 0.0 |
841159 | 13.0 | 14 | 0 | -1.0 |
841160 rows × 4 columns
1
pd.concat([df_all[['loan_amnt', 'installment', 'annual_inc', 'dti', 'loan_status']],df_all.loan_amnt/df_all.annual_inc], axis=1)
loan_amnt | installment | annual_inc | dti | loan_status | 0 | |
---|---|---|---|---|---|---|
0 | 14000 | 470.71 | 88000.00 | 10.02 | 0 | 0.159091 |
1 | 15000 | 516.10 | 98000.00 | 6.15 | 0 | 0.153061 |
2 | 15000 | 476.30 | 63000.00 | 16.51 | 0 | 0.238095 |
3 | 10000 | 321.13 | 102000.00 | 15.55 | 0 | 0.098039 |
4 | 20800 | 706.16 | 81500.00 | 16.73 | 0 | 0.255215 |
5 | 27050 | 885.46 | 55000.00 | 22.87 | 0 | 0.491818 |
6 | 9750 | 333.14 | 26000.00 | 25.12 | 0 | 0.375000 |
7 | 3000 | 100.87 | 25000.00 | 24.68 | 0 | 0.120000 |
8 | 12000 | 407.40 | 40000.00 | 16.94 | 0 | 0.300000 |
9 | 7550 | 266.34 | 28000.00 | 8.40 | 0 | 0.269643 |
10 | 11100 | 384.68 | 90000.00 | 3.73 | 0 | 0.123333 |
11 | 12000 | 373.94 | 96500.00 | 12.61 | 0 | 0.124352 |
12 | 12000 | 398.52 | 130000.00 | 13.03 | 0 | 0.092308 |
13 | 4800 | 157.13 | 39600.00 | 2.49 | 0 | 0.121212 |
14 | 28000 | 872.52 | 325000.00 | 18.55 | 0 | 0.086154 |
15 | 8000 | 261.88 | 33000.00 | 15.75 | 1 | 0.242424 |
16 | 11500 | 323.54 | 32760.00 | 27.06 | 0 | 0.351038 |
17 | 24000 | 814.80 | 100000.00 | 22.18 | 0 | 0.240000 |
18 | 27600 | 730.78 | 73000.00 | 23.13 | 1 | 0.378082 |
19 | 12000 | 392.81 | 60000.00 | 4.62 | 0 | 0.200000 |
20 | 12000 | 368.45 | 105000.00 | 14.05 | 0 | 0.114286 |
21 | 16000 | 500.65 | 98000.00 | 18.21 | 0 | 0.163265 |
22 | 31825 | 852.05 | 70000.00 | 26.49 | 0 | 0.454643 |
23 | 10000 | 332.10 | 41000.00 | 25.79 | 0 | 0.243902 |
24 | 18450 | 630.40 | 65000.00 | 15.84 | 1 | 0.283846 |
25 | 20000 | 444.79 | 80000.00 | 2.69 | 0 | 0.250000 |
26 | 10075 | 377.00 | 55000.00 | 18.84 | 0 | 0.183182 |
27 | 6000 | 196.41 | 67000.00 | 17.61 | 0 | 0.089552 |
28 | 3000 | 111.45 | 110000.00 | 11.24 | 0 | 0.027273 |
29 | 4500 | 165.46 | 105000.00 | 16.23 | 0 | 0.042857 |
30 | 4000 | 141.11 | 84000.00 | 19.80 | 0 | 0.047619 |
31 | 20000 | 488.92 | 72000.00 | 16.42 | 0 | 0.277778 |
32 | 30000 | 765.89 | 120000.00 | 12.54 | 0 | 0.250000 |
33 | 10000 | 232.58 | 25000.00 | 27.03 | 1 | 0.400000 |
34 | 7200 | 235.69 | 70000.00 | 19.20 | 0 | 0.102857 |
35 | 20000 | 683.36 | 80000.00 | 16.70 | 0 | 0.250000 |
36 | 10000 | 237.80 | 60000.00 | 13.56 | 0 | 0.166667 |
37 | 7500 | 233.72 | 295000.00 | 5.04 | 0 | 0.025424 |
38 | 14825 | 537.83 | 175000.00 | 8.07 | 1 | 0.084714 |
39 | 10000 | 321.13 | 45000.00 | 8.91 | 0 | 0.222222 |
40 | 5000 | 169.75 | 70000.00 | 22.56 | 0 | 0.071429 |
41 | 6000 | 182.62 | 115000.00 | 7.37 | 0 | 0.052174 |
42 | 16000 | 498.59 | 112000.00 | 7.39 | 0 | 0.142857 |
43 | 22875 | 781.60 | 50000.00 | 10.83 | 0 | 0.457500 |
44 | 21000 | 654.39 | 110000.00 | 13.68 | 0 | 0.190909 |
45 | 8325 | 291.09 | 65000.00 | 5.71 | 0 | 0.128077 |
46 | 6000 | 192.68 | 70000.00 | 25.14 | 0 | 0.085714 |
47 | 4000 | 125.17 | 36000.00 | 15.47 | 0 | 0.111111 |
48 | 14575 | 494.82 | 41600.00 | 28.25 | 0 | 0.350361 |
49 | 11200 | 380.24 | 38000.00 | 15.51 | 0 | 0.294737 |
50 | 12000 | 412.88 | 50000.00 | 8.19 | 0 | 0.240000 |
51 | 9800 | 320.80 | 40000.00 | 23.79 | 0 | 0.245000 |
52 | 9600 | 314.25 | 33000.00 | 23.85 | 0 | 0.290909 |
53 | 12000 | 407.40 | 60000.00 | 17.30 | 0 | 0.200000 |
54 | 6250 | 226.74 | 75000.00 | 20.25 | 0 | 0.083333 |
55 | 13225 | 451.88 | 30192.00 | 27.98 | 1 | 0.438030 |
56 | 15850 | 559.12 | 59400.00 | 33.22 | 0 | 0.266835 |
57 | 14000 | 438.07 | 87500.00 | 9.82 | 0 | 0.160000 |
58 | 13000 | 417.47 | 102120.00 | 15.85 | 0 | 0.127301 |
59 | 28100 | 752.32 | 67000.00 | 12.59 | 0 | 0.419403 |
60 | 6000 | 199.26 | 34000.00 | 21.14 | 0 | 0.176471 |
61 | 10100 | 383.02 | 45000.00 | 14.11 | 1 | 0.224444 |
62 | 30000 | 1078.12 | 85000.00 | 16.33 | 0 | 0.352941 |
63 | 14000 | 444.55 | 74628.00 | 25.92 | 0 | 0.187597 |
64 | 23675 | 626.85 | 54000.00 | 30.02 | 1 | 0.438426 |
65 | 19200 | 462.94 | 81000.00 | 26.22 | 0 | 0.237037 |
66 | 13000 | 412.80 | 63000.00 | 20.42 | 0 | 0.206349 |
67 | 9950 | 351.00 | 50000.00 | 17.95 | 0 | 0.199000 |
68 | 7500 | 249.08 | 59600.00 | 15.93 | 0 | 0.125839 |
69 | 14000 | 485.18 | 40000.00 | 14.59 | 0 | 0.350000 |
70 | 19750 | 670.51 | 45000.00 | 18.09 | 0 | 0.438889 |
71 | 10000 | 346.56 | 50000.00 | 17.04 | 0 | 0.200000 |
72 | 26400 | 636.54 | 178000.00 | 12.28 | 0 | 0.148315 |
73 | 3000 | 105.83 | 120000.00 | 9.75 | 0 | 0.025000 |
74 | 28000 | 795.79 | 124000.00 | 8.58 | 1 | 0.225806 |
75 | 14400 | 450.58 | 180000.00 | 8.04 | 0 | 0.080000 |
76 | 15000 | 539.06 | 60000.00 | 3.68 | 1 | 0.250000 |
77 | 23000 | 719.68 | 81500.00 | 25.20 | 0 | 0.282209 |
78 | 12000 | 403.47 | 60000.00 | 19.62 | 0 | 0.200000 |
79 | 9000 | 311.90 | 56000.00 | 21.45 | 0 | 0.160714 |
80 | 19125 | 662.79 | 86000.00 | 16.65 | 0 | 0.222384 |
81 | 10000 | 332.10 | 110000.00 | 10.47 | 0 | 0.090909 |
82 | 10600 | 364.71 | 33000.00 | 25.89 | 0 | 0.321212 |
83 | 9450 | 322.89 | 21900.00 | 17.26 | 1 | 0.431507 |
84 | 25000 | 566.91 | 105000.00 | 7.44 | 0 | 0.238095 |
85 | 15600 | 583.74 | 158000.00 | 16.57 | 0 | 0.098734 |
86 | 25000 | 935.48 | 250000.00 | 5.98 | 0 | 0.100000 |
87 | 34475 | 960.02 | 79000.00 | 12.99 | 0 | 0.436392 |
88 | 35000 | 1000.80 | 93500.00 | 26.63 | 0 | 0.374332 |
89 | 4500 | 144.51 | 38000.00 | 20.02 | 0 | 0.118421 |
90 | 16000 | 376.21 | 92000.00 | 21.91 | 0 | 0.173913 |
91 | 10000 | 311.62 | 85000.00 | 12.11 | 0 | 0.117647 |
92 | 11000 | 344.20 | 38000.00 | 21.54 | 0 | 0.289474 |
93 | 12800 | 470.63 | 48000.00 | 26.80 | 0 | 0.266667 |
94 | 8000 | 273.35 | 79000.00 | 24.05 | 0 | 0.101266 |
95 | 12000 | 410.02 | 90000.00 | 14.76 | 0 | 0.133333 |
96 | 16000 | 570.37 | 80456.00 | 17.03 | 0 | 0.198866 |
97 | 5000 | 155.81 | 50000.00 | 4.54 | 0 | 0.100000 |
98 | 5500 | 212.62 | 90000.00 | 9.56 | 0 | 0.061111 |
99 | 20000 | 635.07 | 80000.00 | 4.74 | 0 | 0.250000 |
... | ... | ... | ... | ... | ... | ... |
841060 | 12650 | 391.01 | 105000.00 | 18.05 | 0 | 0.120476 |
841061 | 35000 | 1153.75 | 54000.00 | 25.36 | 0 | 0.648148 |
841062 | 1000 | 33.21 | 34000.00 | 8.98 | 0 | 0.029412 |
841063 | 20000 | 546.15 | 41500.00 | 19.03 | 0 | 0.481928 |
841064 | 7575 | 245.93 | 35928.00 | 21.14 | 0 | 0.210838 |
841065 | 8000 | 261.57 | 75000.00 | 28.00 | 0 | 0.106667 |
841066 | 8000 | 285.59 | 172000.00 | 25.24 | 0 | 0.046512 |
841067 | 23800 | 772.50 | 53000.00 | 31.36 | 0 | 0.449057 |
841068 | 14000 | 283.67 | 50000.00 | 15.67 | 0 | 0.280000 |
841069 | 25000 | 536.36 | 85000.00 | 8.95 | 0 | 0.294118 |
841070 | 10000 | 335.12 | 80000.00 | 6.36 | 0 | 0.125000 |
841071 | 10400 | 298.47 | 45000.00 | 25.84 | 0 | 0.231111 |
841072 | 2500 | 80.59 | 63000.00 | 24.99 | 0 | 0.039683 |
841073 | 6500 | 203.60 | 125000.00 | 7.56 | 0 | 0.052000 |
841074 | 16000 | 415.32 | 79882.37 | 14.19 | 0 | 0.200295 |
841075 | 4200 | 159.21 | 45000.00 | 19.15 | 0 | 0.093333 |
841076 | 2000 | 74.33 | 86086.00 | 24.26 | 0 | 0.023233 |
841077 | 9000 | 292.19 | 55000.00 | 28.39 | 0 | 0.163636 |
841078 | 4800 | 159.41 | 49088.00 | 17.22 | 0 | 0.097784 |
841079 | 21000 | 686.62 | 120000.00 | 27.32 | 0 | 0.175000 |
841080 | 10000 | 301.15 | 100000.00 | 7.96 | 0 | 0.100000 |
841081 | 3000 | 107.10 | 71300.00 | 17.59 | 0 | 0.042076 |
841082 | 15000 | 364.94 | 110000.00 | 22.06 | 0 | 0.136364 |
841083 | 10500 | 328.89 | 68000.00 | 14.03 | 0 | 0.154412 |
841084 | 12200 | 382.14 | 142000.00 | 19.97 | 0 | 0.085915 |
841085 | 10000 | 249.01 | 115016.00 | 16.59 | 0 | 0.086944 |
841086 | 12000 | 375.88 | 38500.00 | 16.95 | 0 | 0.311688 |
841087 | 32000 | 993.20 | 84000.00 | 14.42 | 0 | 0.380952 |
841088 | 2000 | 74.33 | 45760.00 | 27.77 | 0 | 0.043706 |
841089 | 28000 | 843.32 | 70000.00 | 29.92 | 0 | 0.400000 |
841090 | 10000 | 335.12 | 100000.00 | 17.40 | 0 | 0.100000 |
841091 | 5000 | 163.49 | 55000.00 | 2.47 | 0 | 0.090909 |
841092 | 7200 | 289.41 | 43500.00 | 10.12 | 0 | 0.165517 |
841093 | 40000 | 858.18 | 180000.00 | 5.83 | 0 | 0.222222 |
841094 | 7500 | 251.34 | 95000.00 | 6.31 | 0 | 0.078947 |
841095 | 25000 | 662.35 | 130000.00 | 28.62 | 0 | 0.192308 |
841096 | 5000 | 160.03 | 75000.00 | 8.43 | 0 | 0.066667 |
841097 | 16500 | 492.27 | 54000.00 | 23.36 | 0 | 0.305556 |
841098 | 6000 | 211.01 | 80000.00 | 13.25 | 0 | 0.075000 |
841099 | 7600 | 267.27 | 46000.00 | 12.42 | 0 | 0.165217 |
841100 | 5000 | 169.90 | 25000.00 | 19.18 | 0 | 0.200000 |
841101 | 4000 | 125.30 | 50000.00 | 9.22 | 0 | 0.080000 |
841102 | 2000 | 67.96 | 60000.00 | 26.46 | 0 | 0.033333 |
841103 | 4800 | 166.52 | 78000.00 | 25.12 | 0 | 0.061538 |
841104 | 11000 | 341.42 | 32000.00 | 12.98 | 0 | 0.343750 |
841105 | 25000 | 811.62 | 120000.00 | 19.20 | 0 | 0.208333 |
841106 | 6800 | 217.64 | 78000.00 | 3.14 | 0 | 0.087179 |
841107 | 3025 | 101.38 | 65000.00 | 19.77 | 0 | 0.046538 |
841108 | 22000 | 763.18 | 95000.00 | 16.84 | 0 | 0.231579 |
841109 | 10000 | 233.10 | 75000.00 | 25.84 | 0 | 0.133333 |
841110 | 15000 | 490.45 | 175000.00 | 16.07 | 0 | 0.085714 |
841111 | 30000 | 903.45 | 207000.00 | 16.73 | 0 | 0.144928 |
841112 | 1800 | 62.45 | 50000.00 | 16.44 | 0 | 0.036000 |
841113 | 40000 | 1280.20 | 400000.00 | 8.30 | 0 | 0.100000 |
841114 | 7000 | 227.26 | 79000.00 | 13.22 | 0 | 0.088608 |
841115 | 8000 | 257.88 | 55000.00 | 29.48 | 0 | 0.145455 |
841116 | 2800 | 89.62 | 44000.00 | 10.77 | 0 | 0.063636 |
841117 | 8000 | 247.28 | 67000.00 | 20.20 | 0 | 0.119403 |
841118 | 12000 | 257.46 | 63000.00 | 22.60 | 0 | 0.190476 |
841119 | 13000 | 419.05 | 50000.00 | 23.43 | 0 | 0.260000 |
841120 | 5000 | 198.41 | 36000.00 | 38.83 | 0 | 0.138889 |
841121 | 17250 | 615.79 | 40000.00 | 11.43 | 0 | 0.431250 |
841122 | 10000 | 243.29 | 94000.00 | 9.86 | 0 | 0.106383 |
841123 | 7200 | 241.29 | 55000.00 | 32.34 | 0 | 0.130909 |
841124 | 25000 | 582.75 | 64000.00 | 13.71 | 0 | 0.390625 |
841125 | 25000 | 745.85 | 81000.00 | 21.62 | 0 | 0.308642 |
841126 | 11000 | 365.31 | 41500.00 | 12.04 | 0 | 0.265060 |
841127 | 3600 | 128.52 | 40000.00 | 29.53 | 0 | 0.090000 |
841128 | 2100 | 73.86 | 33000.00 | 1.82 | 0 | 0.063636 |
841129 | 25000 | 682.68 | 55000.00 | 23.94 | 0 | 0.454545 |
841130 | 35000 | 1162.34 | 250000.00 | 12.09 | 0 | 0.140000 |
841131 | 10000 | 209.73 | 64000.00 | 20.96 | 0 | 0.156250 |
841132 | 25000 | 682.68 | 25000.00 | 45.18 | 0 | 1.000000 |
841133 | 12000 | 361.38 | 150000.00 | 0.72 | 0 | 0.080000 |
841134 | 2800 | 99.96 | 65000.00 | 27.42 | 0 | 0.043077 |
841135 | 5000 | 169.90 | 42000.00 | 25.51 | 0 | 0.119048 |
841136 | 40000 | 1298.59 | 56000.00 | 22.57 | 0 | 0.714286 |
841137 | 2550 | 85.46 | 67000.00 | 9.05 | 0 | 0.038060 |
841138 | 8000 | 271.84 | 65000.00 | 5.61 | 0 | 0.123077 |
841139 | 21600 | 480.38 | 68000.00 | 20.74 | 0 | 0.317647 |
841140 | 2400 | 75.18 | 117000.00 | 21.79 | 0 | 0.020513 |
841141 | 8100 | 261.10 | 50000.00 | 2.61 | 0 | 0.162000 |
841142 | 4700 | 147.22 | 59000.00 | 19.49 | 0 | 0.079661 |
841143 | 15000 | 490.45 | 113196.00 | 21.87 | 0 | 0.132514 |
841144 | 17925 | 648.58 | 39000.00 | 39.78 | 0 | 0.459615 |
841145 | 12000 | 410.60 | 105000.00 | 0.83 | 0 | 0.114286 |
841146 | 16000 | 531.36 | 175000.00 | 5.51 | 0 | 0.091429 |
841147 | 8500 | 274.00 | 125000.00 | 6.93 | 0 | 0.068000 |
841148 | 12000 | 434.20 | 64000.00 | 31.31 | 0 | 0.187500 |
841149 | 5000 | 195.85 | 40000.00 | 29.82 | 0 | 0.125000 |
841150 | 16000 | 562.68 | 49800.00 | 32.87 | 0 | 0.321285 |
841151 | 4000 | 146.69 | 50000.00 | 13.54 | 0 | 0.080000 |
841152 | 35000 | 1162.34 | 275000.00 | 6.60 | 0 | 0.127273 |
841153 | 12600 | 509.68 | 35000.00 | 31.89 | 0 | 0.360000 |
841154 | 5000 | 193.32 | 40000.00 | 26.37 | 0 | 0.125000 |
841155 | 14000 | 382.30 | 70000.00 | 10.94 | 0 | 0.200000 |
841156 | 13200 | 500.37 | 56160.00 | 12.39 | 0 | 0.235043 |
841157 | 10000 | 404.51 | 33000.00 | 4.40 | 0 | 0.303030 |
841158 | 15000 | 492.24 | 55000.00 | 21.25 | 0 | 0.272727 |
841159 | 25000 | 818.85 | 54778.00 | 12.81 | 0 | 0.456388 |
841160 rows × 6 columns
Observation
- majority of time loan is capped to 50% of annual income with upper limit of 40K
- big cluster of detault are within 25-35K of loan amount
1
2
3
4
%%time
plt.figure(figsize=(16,16))
sns.scatterplot(df_all[df_all.annual_inc < 600000]['annual_inc'], df_all[df_all.annual_inc < 600000]['loan_amnt'], hue=df_all.loan_status)
1
Wall time: 2.61 s
1
2
3
%%time
plt.figure(figsize=(16,16))
sns.scatterplot(df_all[df_all.annual_inc < 200000]['annual_inc'], df_all[df_all.annual_inc < 200000]['loan_amnt'], hue=df_all.loan_status)
1
Wall time: 2.59 s
1
2
3
4
5
6
plt.figure(figsize=(16,16))
#sns.set(style="ticks")
ax=plt.subplot(111)
sns.catplot(x='grade', y='loan_amnt',hue='loan_status',row_order=['A','B','C','D','E','F','G'], data=df_all.sort_values(by='grade'), ax=ax)
plt.close(2)
plt.show()
1
df_all.select_dtypes(include='object').head()
grade | sub_grade | home_ownership | verification_status | purpose | addr_state | |
---|---|---|---|---|---|---|
0 | B | B4 | RENT | Not Verified | debt_consolidation | NC |
1 | C | C2 | RENT | Not Verified | debt_consolidation | NY |
2 | A | A5 | MORTGAGE | Not Verified | debt_consolidation | FL |
3 | B | B1 | MORTGAGE | Not Verified | debt_consolidation | MA |
4 | B | B5 | RENT | Verified | debt_consolidation | NY |
1
2
3
4
5
6
7
8
9
10
11
12
13
14
15
16
def data_preprocess(df):
numerical_attr = [i for i in df.select_dtypes(exclude=['datetime64','object']).columns if i not in ['loan_status']]
print(numerical_attr)
scaler=StandardScaler()
scaler.fit(df[numerical_attr])
df_num=pd.DataFrame(scaler.transform(df[numerical_attr]), index=df[numerical_attr].index, columns=df[numerical_attr].columns)
df_num['loan_status']=df['loan_status']
cat_att= [i for i in df.select_dtypes(include=['object']).columns ]
print(cat_att)
cat_enc = OrdinalEncoder()
cat_enc.fit(df[['grade', 'sub_grade']])
df[['grade', 'sub_grade']]=pd.DataFrame(cat_enc.transform(df[['grade', 'sub_grade']]))
df_cat = pd.get_dummies(df[cat_att])
df = pd.concat([df_num,df_cat],axis=1)
return df
1
2
3
4
5
6
%%time
df_all_process = df_all.copy()
df_all_process = data_preprocess(df_all_process)
cols=[i for i in df_all_process.columns if i not in 'loan_status']
cols.insert(0,'loan_status')
df_all_process = df_all_process[cols]
1
2
3
['loan_amnt', 'term', 'int_rate', 'installment', 'emp_length', 'annual_inc', 'dti', 'delinq_2yrs', 'fico_range_low', 'inq_last_6mths', 'mths_since_last_delinq', 'open_acc', 'pub_rec', 'revol_bal', 'revol_util', 'total_acc', 'mort_acc', 'num_accts_ever_120_pd', 'num_actv_bc_tl', 'num_actv_rev_tl', 'num_bc_sats', 'num_bc_tl', 'num_il_tl', 'num_op_rev_tl', 'num_rev_accts', 'num_rev_tl_bal_gt_0', 'num_sats', 'num_tl_120dpd_2m', 'num_tl_30dpd', 'num_tl_90g_dpd_24m', 'num_tl_op_past_12m', 'pct_tl_nvr_dlq', 'percent_bc_gt_75', 'pub_rec_bankruptcies', 'tax_liens', 'tot_hi_cred_lim', 'total_bal_ex_mort', 'total_bc_limit', 'total_il_high_credit_limit', 'earliest_cr_line_yrs', 'num_un_sats']
['grade', 'sub_grade', 'home_ownership', 'verification_status', 'purpose', 'addr_state']
Wall time: 3.57 s
1
df_all_process.head()
loan_status | loan_amnt | term | int_rate | installment | emp_length | annual_inc | dti | delinq_2yrs | fico_range_low | inq_last_6mths | mths_since_last_delinq | open_acc | pub_rec | revol_bal | revol_util | total_acc | mort_acc | num_accts_ever_120_pd | num_actv_bc_tl | num_actv_rev_tl | num_bc_sats | num_bc_tl | num_il_tl | num_op_rev_tl | num_rev_accts | num_rev_tl_bal_gt_0 | num_sats | num_tl_120dpd_2m | num_tl_30dpd | num_tl_90g_dpd_24m | num_tl_op_past_12m | pct_tl_nvr_dlq | percent_bc_gt_75 | pub_rec_bankruptcies | tax_liens | tot_hi_cred_lim | total_bal_ex_mort | total_bc_limit | total_il_high_credit_limit | earliest_cr_line_yrs | num_un_sats | grade | sub_grade | home_ownership_ANY | home_ownership_MORTGAGE | home_ownership_NONE | home_ownership_OTHER | home_ownership_OWN | home_ownership_RENT | verification_status_Not Verified | verification_status_Source Verified | verification_status_Verified | purpose_car | purpose_credit_card | purpose_debt_consolidation | purpose_educational | purpose_home_improvement | purpose_house | purpose_major_purchase | purpose_medical | purpose_moving | purpose_other | purpose_renewable_energy | purpose_small_business | purpose_vacation | purpose_wedding | addr_state_AK | addr_state_AL | addr_state_AR | addr_state_AZ | addr_state_CA | addr_state_CO | addr_state_CT | addr_state_DC | addr_state_DE | addr_state_FL | addr_state_GA | addr_state_HI | addr_state_IA | addr_state_ID | addr_state_IL | addr_state_IN | addr_state_KS | addr_state_KY | addr_state_LA | addr_state_MA | addr_state_MD | addr_state_ME | addr_state_MI | addr_state_MN | addr_state_MO | addr_state_MS | addr_state_MT | addr_state_NC | addr_state_ND | addr_state_NE | addr_state_NH | addr_state_NJ | addr_state_NM | addr_state_NV | addr_state_NY | addr_state_OH | addr_state_OK | addr_state_OR | addr_state_PA | addr_state_RI | addr_state_SC | addr_state_SD | addr_state_TN | addr_state_TX | addr_state_UT | addr_state_VA | addr_state_VT | addr_state_WA | addr_state_WI | addr_state_WV | addr_state_WY | |
---|---|---|---|---|---|---|---|---|---|---|---|---|---|---|---|---|---|---|---|---|---|---|---|---|---|---|---|---|---|---|---|---|---|---|---|---|---|---|---|---|---|---|---|---|---|---|---|---|---|---|---|---|---|---|---|---|---|---|---|---|---|---|---|---|---|---|---|---|---|---|---|---|---|---|---|---|---|---|---|---|---|---|---|---|---|---|---|---|---|---|---|---|---|---|---|---|---|---|---|---|---|---|---|---|---|---|---|---|---|---|---|---|---|---|---|---|---|---|
0 | 0 | -0.055459 | -0.561572 | -0.143850 | 0.110740 | -0.444194 | 0.189133 | -0.889324 | 0.779871 | -0.799511 | -0.720325 | -1.044451 | -1.051674 | 1.308269 | -0.571128 | 1.195362 | -0.962404 | -0.843467 | -0.379514 | -0.285074 | -0.515008 | -0.597151 | 0.140271 | -0.767586 | -0.968083 | -0.613865 | -0.512817 | -1.057111 | -0.026606 | -0.054514 | -0.173928 | -1.219786 | -1.899928 | 1.501351 | 2.260797 | -0.126956 | -0.806574 | -0.670499 | -0.817243 | -0.323539 | 1.188375 | -0.170197 | 1.0 | 8.0 | 0 | 0 | 0 | 0 | 0 | 1 | 1 | 0 | 0 | 0 | 0 | 1 | 0 | 0 | 0 | 0 | 0 | 0 | 0 | 0 | 0 | 0 | 0 | 0 | 0 | 0 | 0 | 0 | 0 | 0 | 0 | 0 | 0 | 0 | 0 | 0 | 0 | 0 | 0 | 0 | 0 | 0 | 0 | 0 | 0 | 0 | 0 | 0 | 0 | 0 | 1 | 0 | 0 | 0 | 0 | 0 | 0 | 0 | 0 | 0 | 0 | 0 | 0 | 0 | 0 | 0 | 0 | 0 | 0 | 0 | 0 | 0 | 0 | 0 |
1 | 0 | 0.061517 | -0.561572 | 0.202724 | 0.287039 | 1.127142 | 0.342579 | -1.312564 | -0.362172 | 0.659549 | 1.327905 | 0.992706 | 0.811383 | -0.359792 | -0.477234 | -1.273583 | -0.795515 | -0.843467 | -0.379514 | 2.022021 | 0.743729 | 2.913591 | 0.976029 | -1.043251 | 1.536307 | 0.011445 | 0.774460 | 0.823733 | -0.026606 | -0.054514 | -0.173928 | -0.108696 | 0.663821 | -1.066802 | -0.358433 | -0.126956 | -0.798094 | -0.770520 | -0.003519 | -0.800484 | 0.646533 | -0.170197 | 2.0 | 11.0 | 0 | 0 | 0 | 0 | 0 | 1 | 1 | 0 | 0 | 0 | 0 | 1 | 0 | 0 | 0 | 0 | 0 | 0 | 0 | 0 | 0 | 0 | 0 | 0 | 0 | 0 | 0 | 0 | 0 | 0 | 0 | 0 | 0 | 0 | 0 | 0 | 0 | 0 | 0 | 0 | 0 | 0 | 0 | 0 | 0 | 0 | 0 | 0 | 0 | 0 | 0 | 0 | 0 | 0 | 0 | 0 | 0 | 1 | 0 | 0 | 0 | 0 | 0 | 0 | 0 | 0 | 0 | 0 | 0 | 0 | 0 | 0 | 0 | 0 |
2 | 0 | 0.061517 | -0.561572 | -0.988893 | 0.132452 | -0.967972 | -0.194482 | -0.179549 | -0.362172 | -0.799511 | -0.720325 | -1.007148 | -0.679062 | -0.359792 | -0.218626 | 0.876387 | 0.289265 | 1.097274 | 1.971224 | -0.285074 | -0.515008 | -0.597151 | 0.349211 | -0.078423 | -0.512740 | 0.261569 | -0.512817 | -0.680942 | -0.026606 | -0.054514 | -0.173928 | -1.219786 | -0.618053 | 0.574813 | -0.358433 | -0.126956 | 0.682390 | -0.200485 | -0.321305 | -0.165960 | -0.166228 | -0.170197 | 0.0 | 4.0 | 0 | 1 | 0 | 0 | 0 | 0 | 1 | 0 | 0 | 0 | 0 | 1 | 0 | 0 | 0 | 0 | 0 | 0 | 0 | 0 | 0 | 0 | 0 | 0 | 0 | 0 | 0 | 0 | 0 | 0 | 0 | 0 | 1 | 0 | 0 | 0 | 0 | 0 | 0 | 0 | 0 | 0 | 0 | 0 | 0 | 0 | 0 | 0 | 0 | 0 | 0 | 0 | 0 | 0 | 0 | 0 | 0 | 0 | 0 | 0 | 0 | 0 | 0 | 0 | 0 | 0 | 0 | 0 | 0 | 0 | 0 | 0 | 0 | 0 |
3 | 0 | -0.523365 | -0.561572 | -0.824163 | -0.470242 | 0.341474 | 0.403957 | -0.284539 | 1.921915 | -0.799511 | -0.720325 | -1.054813 | -0.492757 | -0.359792 | -0.287761 | -0.358085 | -0.294847 | -0.843467 | 0.404066 | -0.285074 | -0.515008 | -0.597151 | -0.486547 | 0.059409 | -0.512740 | -0.238679 | -0.512817 | -0.492858 | -0.026606 | -0.054514 | -0.173928 | -0.664241 | -2.055670 | 0.574813 | -0.358433 | -0.126956 | -0.651808 | -0.207068 | -0.562051 | -0.110885 | 1.052914 | -0.170197 | 1.0 | 5.0 | 0 | 1 | 0 | 0 | 0 | 0 | 1 | 0 | 0 | 0 | 0 | 1 | 0 | 0 | 0 | 0 | 0 | 0 | 0 | 0 | 0 | 0 | 0 | 0 | 0 | 0 | 0 | 0 | 0 | 0 | 0 | 0 | 0 | 0 | 0 | 0 | 0 | 0 | 0 | 0 | 0 | 0 | 1 | 0 | 0 | 0 | 0 | 0 | 0 | 0 | 0 | 0 | 0 | 0 | 0 | 0 | 0 | 0 | 0 | 0 | 0 | 0 | 0 | 0 | 0 | 0 | 0 | 0 | 0 | 0 | 0 | 0 | 0 | 0 |
4 | 0 | 0.739980 | -0.561572 | 0.001625 | 1.025249 | 1.127142 | 0.089393 | -0.155489 | -0.362172 | -0.313157 | 1.327905 | -0.944976 | 3.233356 | -0.359792 | 0.329447 | 0.060310 | 1.290600 | -0.843467 | 0.404066 | 2.022021 | 5.778681 | 2.211443 | 1.811787 | -1.043251 | 4.723713 | 3.137995 | 5.923569 | 3.268831 | -0.026606 | -0.054514 | -0.173928 | 0.446849 | -0.510232 | 0.110152 | -0.358433 | -0.126956 | -0.741173 | -0.545290 | -0.282785 | -0.980781 | -0.166228 | -0.170197 | 1.0 | 9.0 | 0 | 0 | 0 | 0 | 0 | 1 | 0 | 0 | 1 | 0 | 0 | 1 | 0 | 0 | 0 | 0 | 0 | 0 | 0 | 0 | 0 | 0 | 0 | 0 | 0 | 0 | 0 | 0 | 0 | 0 | 0 | 0 | 0 | 0 | 0 | 0 | 0 | 0 | 0 | 0 | 0 | 0 | 0 | 0 | 0 | 0 | 0 | 0 | 0 | 0 | 0 | 0 | 0 | 0 | 0 | 0 | 0 | 1 | 0 | 0 | 0 | 0 | 0 | 0 | 0 | 0 | 0 | 0 | 0 | 0 | 0 | 0 | 0 | 0 |
1
df_all_process.loan_status.value_counts()
1
2
3
0 664574
1 176586
Name: loan_status, dtype: int64
1
df_all_process.to_csv('../data/processed/data_for_modeling.csv', index=False)
1
df_all_process.describe().transpose()
count | mean | std | min | 25% | 50% | 75% | max | |
---|---|---|---|---|---|---|---|---|
loan_status | 841160.0 | 2.099315e-01 | 0.407260 | 0.000000 | 0.000000 | 0.000000 | 0.000000 | 1.000000 |
loan_amnt | 841160.0 | -1.824488e-14 | 1.000001 | -1.576152 | -0.757318 | -0.289412 | 0.646399 | 2.985926 |
term | 841160.0 | -8.095091e-14 | 1.000001 | -0.561572 | -0.561572 | -0.561572 | -0.561572 | 1.780717 |
int_rate | 841160.0 | -4.712775e-14 | 1.000001 | -1.754779 | -0.755704 | -0.088228 | 0.592085 | 3.736926 |
installment | 841160.0 | -7.374048e-15 | 1.000001 | -1.698391 | -0.725932 | -0.233157 | 0.548865 | 4.941888 |
emp_length | 841160.0 | 4.837188e-14 | 1.000001 | -1.491750 | -0.967972 | 0.079585 | 1.127142 | 1.127142 |
annual_inc | 841160.0 | -3.648181e-15 | 1.000001 | -1.161191 | -0.455340 | -0.163793 | 0.219822 | 145.379705 |
dti | 841160.0 | 1.790886e-15 | 1.000001 | -2.094519 | -0.687000 | -0.058155 | 0.637402 | 107.269811 |
delinq_2yrs | 841160.0 | -7.785799e-16 | 1.000001 | -0.362172 | -0.362172 | -0.362172 | -0.362172 | 44.177518 |
fico_range_low | 841160.0 | 3.698786e-15 | 1.000001 | -1.123746 | -0.799511 | -0.313157 | 0.497431 | 4.874609 |
inq_last_6mths | 841160.0 | 3.092004e-13 | 1.000001 | -0.720325 | -0.720325 | -0.720325 | 0.303790 | 7.472595 |
mths_since_last_delinq | 841160.0 | 2.568070e-13 | 1.000001 | -1.077609 | -1.013365 | 0.992706 | 0.992706 | 0.992706 |
open_acc | 841160.0 | 9.152326e-14 | 1.000001 | -2.169507 | -0.679062 | -0.120145 | 0.438772 | 14.598000 |
pub_rec | 841160.0 | 9.092064e-14 | 1.000001 | -0.359792 | -0.359792 | -0.359792 | -0.359792 | 143.093446 |
revol_bal | 841160.0 | -2.212570e-16 | 1.000001 | -0.738891 | -0.460667 | -0.225408 | 0.164506 | 116.185040 |
revol_util | 841160.0 | -1.241277e-14 | 1.000001 | -2.197366 | -0.743340 | 0.023027 | 0.772824 | 34.766386 |
total_acc | 841160.0 | -9.577979e-15 | 1.000001 | -1.963739 | -0.712070 | -0.127958 | 0.539599 | 12.555621 |
mort_acc | 841160.0 | -3.952062e-14 | 1.000001 | -0.843467 | -0.843467 | -0.358282 | 0.612089 | 23.900989 |
num_accts_ever_120_pd | 841160.0 | 5.975383e-13 | 1.000001 | -0.379514 | -0.379514 | -0.379514 | -0.379514 | 39.583025 |
num_actv_bc_tl | 841160.0 | -2.400621e-14 | 1.000001 | -1.669330 | -0.746492 | -0.285074 | 0.637764 | 13.557494 |
num_actv_rev_tl | 841160.0 | -3.608840e-15 | 1.000001 | -1.773746 | -0.829693 | -0.200324 | 0.429045 | 16.163267 |
num_bc_sats | 841160.0 | 4.980532e-15 | 1.000001 | -1.650373 | -0.597151 | -0.246077 | 0.456072 | 20.467299 |
num_bc_tl | 841160.0 | -7.431180e-14 | 1.000001 | -1.740184 | -0.695487 | -0.277608 | 0.558150 | 12.885579 |
num_il_tl | 841160.0 | -2.879555e-13 | 1.000001 | -1.181083 | -0.629753 | -0.216256 | 0.335074 | 19.493788 |
num_op_rev_tl | 841160.0 | -4.690038e-14 | 1.000001 | -1.878771 | -0.740412 | -0.285068 | 0.397948 | 17.017994 |
num_rev_accts | 841160.0 | 1.200053e-14 | 1.000001 | -1.864485 | -0.738927 | -0.238679 | 0.511693 | 14.143450 |
num_rev_tl_bal_gt_0 | 841160.0 | -8.889964e-15 | 1.000001 | -1.800094 | -0.834636 | -0.190998 | 0.452641 | 12.681775 |
num_sats | 841160.0 | -7.072421e-14 | 1.000001 | -2.185618 | -0.680942 | -0.116689 | 0.447564 | 14.741980 |
num_tl_120dpd_2m | 841160.0 | -3.982046e-14 | 1.000001 | -0.026606 | -0.026606 | -0.026606 | -0.026606 | 198.611976 |
num_tl_30dpd | 841160.0 | 1.857497e-14 | 1.000001 | -0.054514 | -0.054514 | -0.054514 | -0.054514 | 64.712016 |
num_tl_90g_dpd_24m | 841160.0 | 1.078840e-13 | 1.000001 | -0.173928 | -0.173928 | -0.173928 | -0.173928 | 79.461950 |
num_tl_op_past_12m | 841160.0 | -8.425055e-13 | 1.000001 | -1.219786 | -0.664241 | -0.108696 | 0.446849 | 16.557654 |
pct_tl_nvr_dlq | 841160.0 | -2.180333e-13 | 1.000001 | -11.316317 | -0.294590 | 0.424219 | 0.663821 | 0.663821 |
percent_bc_gt_75 | 841160.0 | 3.107445e-14 | 1.000001 | -1.281047 | -0.933247 | 0.110152 | 0.805752 | 1.501351 |
pub_rec_bankruptcies | 841160.0 | 3.646427e-13 | 1.000001 | -0.358433 | -0.358433 | -0.358433 | -0.358433 | 31.072323 |
tax_liens | 841160.0 | -6.273211e-15 | 1.000001 | -0.126956 | -0.126956 | -0.126956 | -0.126956 | 217.666488 |
tot_hi_cred_lim | 841160.0 | 9.011056e-16 | 1.000001 | -0.991507 | -0.698878 | -0.338932 | 0.428697 | 57.090577 |
total_bal_ex_mort | 841160.0 | 6.637531e-15 | 1.000001 | -1.051933 | -0.599380 | -0.255222 | 0.269163 | 72.508600 |
total_bc_limit | 841160.0 | -4.557264e-15 | 1.000001 | -1.005026 | -0.643905 | -0.302045 | 0.295008 | 52.224115 |
total_il_high_credit_limit | 841160.0 | -2.975681e-14 | 1.000001 | -0.980781 | -0.620187 | -0.235530 | 0.322188 | 49.548301 |
earliest_cr_line_yrs | 841160.0 | -4.399923e-14 | 1.000001 | -1.791752 | -0.708070 | -0.166228 | 0.511073 | 7.419549 |
num_un_sats | 841160.0 | -1.196969e-13 | 1.000001 | -0.170197 | -0.170197 | -0.170197 | -0.170197 | 101.938083 |
grade | 841160.0 | 1.813964e+00 | 1.315627 | 0.000000 | 1.000000 | 2.000000 | 3.000000 | 6.000000 |
sub_grade | 841160.0 | 1.103449e+01 | 6.529283 | 0.000000 | 6.000000 | 10.000000 | 15.000000 | 34.000000 |
home_ownership_ANY | 841160.0 | 1.343383e-04 | 0.011590 | 0.000000 | 0.000000 | 0.000000 | 0.000000 | 1.000000 |
home_ownership_MORTGAGE | 841160.0 | 5.006871e-01 | 0.500000 | 0.000000 | 0.000000 | 1.000000 | 1.000000 | 1.000000 |
home_ownership_NONE | 841160.0 | 5.111988e-05 | 0.007150 | 0.000000 | 0.000000 | 0.000000 | 0.000000 | 1.000000 |
home_ownership_OTHER | 841160.0 | 5.468639e-05 | 0.007395 | 0.000000 | 0.000000 | 0.000000 | 0.000000 | 1.000000 |
home_ownership_OWN | 841160.0 | 1.035784e-01 | 0.304713 | 0.000000 | 0.000000 | 0.000000 | 0.000000 | 1.000000 |
home_ownership_RENT | 841160.0 | 3.954943e-01 | 0.488957 | 0.000000 | 0.000000 | 0.000000 | 1.000000 | 1.000000 |
verification_status_Not Verified | 841160.0 | 3.021744e-01 | 0.459201 | 0.000000 | 0.000000 | 0.000000 | 1.000000 | 1.000000 |
verification_status_Source Verified | 841160.0 | 3.695385e-01 | 0.482680 | 0.000000 | 0.000000 | 0.000000 | 1.000000 | 1.000000 |
verification_status_Verified | 841160.0 | 3.282871e-01 | 0.469590 | 0.000000 | 0.000000 | 0.000000 | 1.000000 | 1.000000 |
purpose_car | 841160.0 | 9.634315e-03 | 0.097681 | 0.000000 | 0.000000 | 0.000000 | 0.000000 | 1.000000 |
purpose_credit_card | 841160.0 | 2.195635e-01 | 0.413951 | 0.000000 | 0.000000 | 0.000000 | 0.000000 | 1.000000 |
purpose_debt_consolidation | 841160.0 | 5.958201e-01 | 0.490733 | 0.000000 | 0.000000 | 1.000000 | 1.000000 | 1.000000 |
purpose_educational | 841160.0 | 1.188834e-06 | 0.001090 | 0.000000 | 0.000000 | 0.000000 | 0.000000 | 1.000000 |
purpose_home_improvement | 841160.0 | 6.195016e-02 | 0.241065 | 0.000000 | 0.000000 | 0.000000 | 0.000000 | 1.000000 |
purpose_house | 841160.0 | 4.802891e-03 | 0.069136 | 0.000000 | 0.000000 | 0.000000 | 0.000000 | 1.000000 |
purpose_major_purchase | 841160.0 | 1.983214e-02 | 0.139423 | 0.000000 | 0.000000 | 0.000000 | 0.000000 | 1.000000 |
purpose_medical | 841160.0 | 1.052475e-02 | 0.102049 | 0.000000 | 0.000000 | 0.000000 | 0.000000 | 1.000000 |
purpose_moving | 841160.0 | 6.657473e-03 | 0.081321 | 0.000000 | 0.000000 | 0.000000 | 0.000000 | 1.000000 |
purpose_other | 841160.0 | 5.217794e-02 | 0.222386 | 0.000000 | 0.000000 | 0.000000 | 0.000000 | 1.000000 |
purpose_renewable_energy | 841160.0 | 6.491036e-04 | 0.025469 | 0.000000 | 0.000000 | 0.000000 | 0.000000 | 1.000000 |
purpose_small_business | 841160.0 | 1.064839e-02 | 0.102640 | 0.000000 | 0.000000 | 0.000000 | 0.000000 | 1.000000 |
purpose_vacation | 841160.0 | 6.161729e-03 | 0.078255 | 0.000000 | 0.000000 | 0.000000 | 0.000000 | 1.000000 |
purpose_wedding | 841160.0 | 1.576395e-03 | 0.039673 | 0.000000 | 0.000000 | 0.000000 | 0.000000 | 1.000000 |
addr_state_AK | 841160.0 | 2.559561e-03 | 0.050527 | 0.000000 | 0.000000 | 0.000000 | 0.000000 | 1.000000 |
addr_state_AL | 841160.0 | 1.256122e-02 | 0.111371 | 0.000000 | 0.000000 | 0.000000 | 0.000000 | 1.000000 |
addr_state_AR | 841160.0 | 7.481335e-03 | 0.086171 | 0.000000 | 0.000000 | 0.000000 | 0.000000 | 1.000000 |
addr_state_AZ | 841160.0 | 2.435922e-02 | 0.154162 | 0.000000 | 0.000000 | 0.000000 | 0.000000 | 1.000000 |
addr_state_CA | 841160.0 | 1.501403e-01 | 0.357209 | 0.000000 | 0.000000 | 0.000000 | 0.000000 | 1.000000 |
addr_state_CO | 841160.0 | 2.276856e-02 | 0.149165 | 0.000000 | 0.000000 | 0.000000 | 0.000000 | 1.000000 |
addr_state_CT | 841160.0 | 1.397475e-02 | 0.117386 | 0.000000 | 0.000000 | 0.000000 | 0.000000 | 1.000000 |
addr_state_DC | 841160.0 | 2.640401e-03 | 0.051317 | 0.000000 | 0.000000 | 0.000000 | 0.000000 | 1.000000 |
addr_state_DE | 841160.0 | 2.772362e-03 | 0.052580 | 0.000000 | 0.000000 | 0.000000 | 0.000000 | 1.000000 |
addr_state_FL | 841160.0 | 7.018047e-02 | 0.255451 | 0.000000 | 0.000000 | 0.000000 | 0.000000 | 1.000000 |
addr_state_GA | 841160.0 | 3.174426e-02 | 0.175319 | 0.000000 | 0.000000 | 0.000000 | 0.000000 | 1.000000 |
addr_state_HI | 841160.0 | 5.251082e-03 | 0.072274 | 0.000000 | 0.000000 | 0.000000 | 0.000000 | 1.000000 |
addr_state_IA | 841160.0 | 2.377669e-06 | 0.001542 | 0.000000 | 0.000000 | 0.000000 | 0.000000 | 1.000000 |
addr_state_ID | 841160.0 | 7.382662e-04 | 0.027161 | 0.000000 | 0.000000 | 0.000000 | 0.000000 | 1.000000 |
addr_state_IL | 841160.0 | 3.790955e-02 | 0.190978 | 0.000000 | 0.000000 | 0.000000 | 0.000000 | 1.000000 |
addr_state_IN | 841160.0 | 1.618479e-02 | 0.126186 | 0.000000 | 0.000000 | 0.000000 | 0.000000 | 1.000000 |
addr_state_KS | 841160.0 | 8.450235e-03 | 0.091536 | 0.000000 | 0.000000 | 0.000000 | 0.000000 | 1.000000 |
addr_state_KY | 841160.0 | 9.532075e-03 | 0.097166 | 0.000000 | 0.000000 | 0.000000 | 0.000000 | 1.000000 |
addr_state_LA | 841160.0 | 1.170408e-02 | 0.107550 | 0.000000 | 0.000000 | 0.000000 | 0.000000 | 1.000000 |
addr_state_MA | 841160.0 | 2.248680e-02 | 0.148260 | 0.000000 | 0.000000 | 0.000000 | 0.000000 | 1.000000 |
addr_state_MD | 841160.0 | 2.304674e-02 | 0.150052 | 0.000000 | 0.000000 | 0.000000 | 0.000000 | 1.000000 |
addr_state_ME | 841160.0 | 9.570117e-04 | 0.030921 | 0.000000 | 0.000000 | 0.000000 | 0.000000 | 1.000000 |
addr_state_MI | 841160.0 | 2.609611e-02 | 0.159421 | 0.000000 | 0.000000 | 0.000000 | 0.000000 | 1.000000 |
addr_state_MN | 841160.0 | 1.797874e-02 | 0.132874 | 0.000000 | 0.000000 | 0.000000 | 0.000000 | 1.000000 |
addr_state_MO | 841160.0 | 1.563198e-02 | 0.124047 | 0.000000 | 0.000000 | 0.000000 | 0.000000 | 1.000000 |
addr_state_MS | 841160.0 | 4.326169e-03 | 0.065631 | 0.000000 | 0.000000 | 0.000000 | 0.000000 | 1.000000 |
addr_state_MT | 841160.0 | 2.894812e-03 | 0.053726 | 0.000000 | 0.000000 | 0.000000 | 0.000000 | 1.000000 |
addr_state_NC | 841160.0 | 2.855105e-02 | 0.166541 | 0.000000 | 0.000000 | 0.000000 | 0.000000 | 1.000000 |
addr_state_ND | 841160.0 | 8.381283e-04 | 0.028938 | 0.000000 | 0.000000 | 0.000000 | 0.000000 | 1.000000 |
addr_state_NE | 841160.0 | 1.941367e-03 | 0.044018 | 0.000000 | 0.000000 | 0.000000 | 0.000000 | 1.000000 |
addr_state_NH | 841160.0 | 4.651909e-03 | 0.068046 | 0.000000 | 0.000000 | 0.000000 | 0.000000 | 1.000000 |
addr_state_NJ | 841160.0 | 3.563769e-02 | 0.185385 | 0.000000 | 0.000000 | 0.000000 | 0.000000 | 1.000000 |
addr_state_NM | 841160.0 | 5.682629e-03 | 0.075169 | 0.000000 | 0.000000 | 0.000000 | 0.000000 | 1.000000 |
addr_state_NV | 841160.0 | 1.521708e-02 | 0.122415 | 0.000000 | 0.000000 | 0.000000 | 0.000000 | 1.000000 |
addr_state_NY | 841160.0 | 8.111774e-02 | 0.273016 | 0.000000 | 0.000000 | 0.000000 | 0.000000 | 1.000000 |
addr_state_OH | 841160.0 | 3.233511e-02 | 0.176889 | 0.000000 | 0.000000 | 0.000000 | 0.000000 | 1.000000 |
addr_state_OK | 841160.0 | 9.060107e-03 | 0.094752 | 0.000000 | 0.000000 | 0.000000 | 0.000000 | 1.000000 |
addr_state_OR | 841160.0 | 1.271577e-02 | 0.112045 | 0.000000 | 0.000000 | 0.000000 | 0.000000 | 1.000000 |
addr_state_PA | 841160.0 | 3.381640e-02 | 0.180756 | 0.000000 | 0.000000 | 0.000000 | 0.000000 | 1.000000 |
addr_state_RI | 841160.0 | 4.278615e-03 | 0.065271 | 0.000000 | 0.000000 | 0.000000 | 0.000000 | 1.000000 |
addr_state_SC | 841160.0 | 1.159946e-02 | 0.107074 | 0.000000 | 0.000000 | 0.000000 | 0.000000 | 1.000000 |
addr_state_SD | 841160.0 | 2.101859e-03 | 0.045798 | 0.000000 | 0.000000 | 0.000000 | 0.000000 | 1.000000 |
addr_state_TN | 841160.0 | 1.508512e-02 | 0.121892 | 0.000000 | 0.000000 | 0.000000 | 0.000000 | 1.000000 |
addr_state_TX | 841160.0 | 8.111418e-02 | 0.273011 | 0.000000 | 0.000000 | 0.000000 | 0.000000 | 1.000000 |
addr_state_UT | 841160.0 | 7.937848e-03 | 0.088740 | 0.000000 | 0.000000 | 0.000000 | 0.000000 | 1.000000 |
addr_state_VA | 841160.0 | 2.875909e-02 | 0.167129 | 0.000000 | 0.000000 | 0.000000 | 0.000000 | 1.000000 |
addr_state_VT | 841160.0 | 1.915212e-03 | 0.043721 | 0.000000 | 0.000000 | 0.000000 | 0.000000 | 1.000000 |
addr_state_WA | 841160.0 | 2.225617e-02 | 0.147516 | 0.000000 | 0.000000 | 0.000000 | 0.000000 | 1.000000 |
addr_state_WI | 841160.0 | 1.282277e-02 | 0.112509 | 0.000000 | 0.000000 | 0.000000 | 0.000000 | 1.000000 |
addr_state_WV | 841160.0 | 3.943364e-03 | 0.062672 | 0.000000 | 0.000000 | 0.000000 | 0.000000 | 1.000000 |
addr_state_WY | 841160.0 | 2.248086e-03 | 0.047361 | 0.000000 | 0.000000 | 0.000000 | 0.000000 | 1.000000 |
1
2
3
x_train, x_test,y_train,y_test = train_test_split(df_all_process.drop('loan_status', axis=1),\
df_all_process.loan_status,test_size=.2,\
shuffle=True,stratify=df_all_process.loan_status,random_state=20)
1
df_all_process.loan_status.value_counts()[1]/(df_all_process.loan_status.value_counts()[1]+df_all_process.loan_status.value_counts()[0])
1
0.20993152313471872
1
y_train.value_counts()[1]/(y_train.value_counts()[1]+y_train.value_counts()[0])
1
0.2099318203433354
1
y_test.value_counts()[1]/(y_test.value_counts()[1]+y_test.value_counts()[0])
1
0.20993033430025204
LogisticRegression
1
2
3
4
5
6
7
8
%%time
param_grid = [
{'penalty':['l2', 'l1'],
'C': [.01,.1,1]}
]
logiReg = LogisticRegression()
lr_gridSearch = GridSearchCV(logiReg,param_grid,cv=5,verbose=10, n_jobs=4)
1
Wall time: 0 ns
1
2
%%time
lr_gridSearch.fit(x_train,y_train)
1
2
3
4
5
6
7
8
9
10
11
12
13
14
15
16
17
18
19
20
21
22
23
24
25
26
27
28
29
30
31
32
Fitting 5 folds for each of 6 candidates, totalling 30 fits
[Parallel(n_jobs=4)]: Using backend LokyBackend with 4 concurrent workers.
[Parallel(n_jobs=4)]: Done 5 tasks | elapsed: 1.1min
[Parallel(n_jobs=4)]: Done 10 tasks | elapsed: 7.2min
[Parallel(n_jobs=4)]: Done 17 tasks | elapsed: 134.0min
[Parallel(n_jobs=4)]: Done 27 out of 30 | elapsed: 158.2min remaining: 17.6min
[Parallel(n_jobs=4)]: Done 30 out of 30 | elapsed: 160.5min finished
C:\Anaconda3\lib\site-packages\sklearn\linear_model\logistic.py:432: FutureWarning: Default solver will be changed to 'lbfgs' in 0.22. Specify a solver to silence this warning.
FutureWarning)
Wall time: 2h 40min 47s
GridSearchCV(cv=5, error_score='raise-deprecating',
estimator=LogisticRegression(C=1.0, class_weight=None, dual=False,
fit_intercept=True,
intercept_scaling=1, l1_ratio=None,
max_iter=100, multi_class='warn',
n_jobs=None, penalty='l2',
random_state=None, solver='warn',
tol=0.0001, verbose=0,
warm_start=False),
iid='warn', n_jobs=4,
param_grid=[{'C': [0.01, 0.1, 1], 'penalty': ['l2', 'l1']}],
pre_dispatch='2*n_jobs', refit=True, return_train_score=False,
scoring=None, verbose=10)
1
2
#pickle.dump(lr_gridSearch,open('../models/lr_gridSearch.pkl','wb'))
lr_gridSearch=pickle.load(open('../models/lr_gridSearch.pkl','rb'))
1
lr_gridSearch.predict_proba(x_test)
1
2
3
4
5
6
7
array([[0.8020942 , 0.1979058 ],
[0.77695954, 0.22304046],
[0.87419737, 0.12580263],
...,
[0.40202311, 0.59797689],
[0.9310297 , 0.0689703 ],
[0.85267109, 0.14732891]])
LightGBM
1
2
lgb_train = lgb.Dataset( x_train,label=y_train)
lgb_test = lgb.Dataset(x_test,label=y_test, reference=lgb_train)
1
2
3
4
5
6
7
8
9
10
11
%%time
lgb_classifier = lgb.LGBMClassifier(objective='binary', n_jobs=4, silent=False)
lgb_param = [
{
#'num_leaves': [31,7],
'n_estimators': [100,200],
'learning_rate': [.01,.1]
}
]
lgb_score = GridSearchCV(lgb_classifier,lgb_param,cv=5,verbose=10)
1
Wall time: 0 ns
1
2
%%time
lgb_score.fit(x_train,y_train)
1
2
3
4
5
6
7
8
9
10
11
12
13
14
15
16
17
18
19
20
21
22
23
24
25
26
27
28
29
30
31
32
33
34
35
36
37
38
39
40
41
42
43
44
45
46
47
48
49
50
51
52
53
54
55
56
57
58
59
60
61
62
63
64
65
66
67
68
69
70
71
72
73
74
75
76
77
78
79
80
81
82
83
84
85
86
87
88
89
90
91
92
93
94
95
96
97
98
99
100
101
102
103
104
105
106
107
108
109
110
111
112
113
114
115
116
117
118
119
120
121
Fitting 5 folds for each of 4 candidates, totalling 20 fits
[Parallel(n_jobs=1)]: Using backend SequentialBackend with 1 concurrent workers.
[CV] learning_rate=0.01, n_estimators=100 ............................
[CV] learning_rate=0.01, n_estimators=100, score=0.790, total= 8.1s
[Parallel(n_jobs=1)]: Done 1 out of 1 | elapsed: 8.0s remaining: 0.0s
[CV] learning_rate=0.01, n_estimators=100 ............................
[CV] learning_rate=0.01, n_estimators=100, score=0.790, total= 8.4s
[Parallel(n_jobs=1)]: Done 2 out of 2 | elapsed: 16.5s remaining: 0.0s
[CV] learning_rate=0.01, n_estimators=100 ............................
[CV] learning_rate=0.01, n_estimators=100, score=0.790, total= 7.9s
[Parallel(n_jobs=1)]: Done 3 out of 3 | elapsed: 24.4s remaining: 0.0s
[CV] learning_rate=0.01, n_estimators=100 ............................
[CV] learning_rate=0.01, n_estimators=100, score=0.790, total= 8.0s
[Parallel(n_jobs=1)]: Done 4 out of 4 | elapsed: 32.4s remaining: 0.0s
[CV] learning_rate=0.01, n_estimators=100 ............................
[CV] learning_rate=0.01, n_estimators=100, score=0.790, total= 8.1s
[Parallel(n_jobs=1)]: Done 5 out of 5 | elapsed: 40.6s remaining: 0.0s
[CV] learning_rate=0.01, n_estimators=200 ............................
[CV] learning_rate=0.01, n_estimators=200, score=0.792, total= 13.7s
[Parallel(n_jobs=1)]: Done 6 out of 6 | elapsed: 54.3s remaining: 0.0s
[CV] learning_rate=0.01, n_estimators=200 ............................
[CV] learning_rate=0.01, n_estimators=200, score=0.792, total= 14.1s
[Parallel(n_jobs=1)]: Done 7 out of 7 | elapsed: 1.1min remaining: 0.0s
[CV] learning_rate=0.01, n_estimators=200 ............................
[CV] learning_rate=0.01, n_estimators=200, score=0.792, total= 14.9s
[Parallel(n_jobs=1)]: Done 8 out of 8 | elapsed: 1.4min remaining: 0.0s
[CV] learning_rate=0.01, n_estimators=200 ............................
[CV] learning_rate=0.01, n_estimators=200, score=0.792, total= 13.9s
[Parallel(n_jobs=1)]: Done 9 out of 9 | elapsed: 1.6min remaining: 0.0s
[CV] learning_rate=0.01, n_estimators=200 ............................
[CV] learning_rate=0.01, n_estimators=200, score=0.792, total= 14.8s
[CV] learning_rate=0.1, n_estimators=100 .............................
[CV] . learning_rate=0.1, n_estimators=100, score=0.797, total= 7.5s
[CV] learning_rate=0.1, n_estimators=100 .............................
[CV] . learning_rate=0.1, n_estimators=100, score=0.797, total= 8.8s
[CV] learning_rate=0.1, n_estimators=100 .............................
[CV] . learning_rate=0.1, n_estimators=100, score=0.797, total= 7.5s
[CV] learning_rate=0.1, n_estimators=100 .............................
[CV] . learning_rate=0.1, n_estimators=100, score=0.797, total= 7.7s
[CV] learning_rate=0.1, n_estimators=100 .............................
[CV] . learning_rate=0.1, n_estimators=100, score=0.797, total= 7.5s
[CV] learning_rate=0.1, n_estimators=200 .............................
[CV] . learning_rate=0.1, n_estimators=200, score=0.798, total= 10.6s
[CV] learning_rate=0.1, n_estimators=200 .............................
[CV] . learning_rate=0.1, n_estimators=200, score=0.797, total= 10.4s
[CV] learning_rate=0.1, n_estimators=200 .............................
[CV] . learning_rate=0.1, n_estimators=200, score=0.798, total= 10.7s
[CV] learning_rate=0.1, n_estimators=200 .............................
[CV] . learning_rate=0.1, n_estimators=200, score=0.797, total= 10.8s
[CV] learning_rate=0.1, n_estimators=200 .............................
[CV] . learning_rate=0.1, n_estimators=200, score=0.797, total= 10.6s
[Parallel(n_jobs=1)]: Done 20 out of 20 | elapsed: 3.4min finished
Wall time: 3min 36s
GridSearchCV(cv=5, error_score='raise-deprecating',
estimator=LGBMClassifier(boosting_type='gbdt', class_weight=None,
colsample_bytree=1.0,
importance_type='split',
learning_rate=0.1, max_depth=-1,
min_child_samples=20,
min_child_weight=0.001,
min_split_gain=0.0, n_estimators=100,
n_jobs=4, num_leaves=31,
objective='binary', random_state=None,
reg_alpha=0.0, reg_lambda=0.0,
silent=False, subsample=1.0,
subsample_for_bin=200000,
subsample_freq=0),
iid='warn', n_jobs=None,
param_grid=[{'learning_rate': [0.01, 0.1],
'n_estimators': [100, 200]}],
pre_dispatch='2*n_jobs', refit=True, return_train_score=False,
scoring=None, verbose=10)
1
2
#pickle.dump(lgb_score,open('../models/lgb_score.pkl','wb'))
lgb_score=pickle.load(open('../models/lgb_score.pkl','rb'))
1
lgb_score.best_score_
1
0.797482048599553
1
lr_gridSearch.best_score_
1
0.7945545437253316
1
lr_gridSearch.predict_proba(x_test)
1
2
3
4
5
6
7
array([[0.8020942 , 0.1979058 ],
[0.77695954, 0.22304046],
[0.87419737, 0.12580263],
...,
[0.40202311, 0.59797689],
[0.9310297 , 0.0689703 ],
[0.85267109, 0.14732891]])
1
lgb_score.predict_proba(x_test)
1
2
3
4
5
6
7
array([[0.84439808, 0.15560192],
[0.75799806, 0.24200194],
[0.89348039, 0.10651961],
...,
[0.32789187, 0.67210813],
[0.94063322, 0.05936678],
[0.62461911, 0.37538089]])
1
2
plot_roc_curve(lr_gridSearch, x_test,y_test)
plot_roc_curve(lgb_score, x_test,y_test)
1
<sklearn.metrics._plot.roc_curve.RocCurveDisplay at 0x263cc723e80>
1
2
3
4
5
6
7
8
9
10
11
12
%%time
lgb_classifier_tuned = lgb.LGBMClassifier(objective='binary', n_jobs=4, silent=False)
lgb_param_tuned = [
{
'num_leaves': [21,31,41],
'boosting' :['gbdt','dart'],
'n_estimators': [100,200,250]
#'learning_rate': [.01,.1]
}
]
lgb_score_tuned = GridSearchCV(lgb_classifier_tuned,lgb_param_tuned,cv=5,verbose=10)
1
Wall time: 0 ns
1
2
%%time
lgb_score_tuned.fit(x_train,y_train)
1
2
3
4
5
6
7
8
9
10
11
12
13
14
15
16
17
18
19
20
21
22
23
24
25
26
27
28
29
30
31
32
33
34
35
36
37
38
39
40
41
42
43
44
45
46
47
48
49
50
51
52
53
54
55
56
57
58
59
60
61
62
63
64
65
66
67
68
69
70
71
72
73
74
75
76
77
78
79
80
81
82
83
84
85
86
87
88
89
90
91
92
93
94
95
96
97
98
99
100
101
102
103
104
105
106
107
108
109
110
111
112
113
114
115
116
117
118
119
120
121
122
123
124
125
126
127
128
129
130
131
132
133
134
135
136
137
138
139
140
141
142
143
144
145
146
147
148
149
150
151
152
153
154
155
156
157
158
159
160
161
162
163
164
165
166
167
168
169
170
171
172
173
174
175
176
177
178
179
180
181
182
183
184
185
186
187
188
189
190
191
192
193
194
195
196
197
198
199
200
201
202
203
204
205
206
207
208
209
210
211
212
213
214
215
216
217
218
219
220
221
222
223
224
225
226
227
228
229
230
Fitting 5 folds for each of 18 candidates, totalling 90 fits
[CV] boosting=gbdt, n_estimators=100, num_leaves=21 ..................
[Parallel(n_jobs=1)]: Using backend SequentialBackend with 1 concurrent workers.
[CV] boosting=gbdt, n_estimators=100, num_leaves=21, score=0.797, total= 20.7s
[CV] boosting=gbdt, n_estimators=100, num_leaves=21 ..................
[Parallel(n_jobs=1)]: Done 1 out of 1 | elapsed: 20.7s remaining: 0.0s
[CV] boosting=gbdt, n_estimators=100, num_leaves=21, score=0.796, total= 17.0s
[CV] boosting=gbdt, n_estimators=100, num_leaves=21 ..................
[Parallel(n_jobs=1)]: Done 2 out of 2 | elapsed: 37.7s remaining: 0.0s
[CV] boosting=gbdt, n_estimators=100, num_leaves=21, score=0.797, total= 7.0s
[CV] boosting=gbdt, n_estimators=100, num_leaves=21 ..................
[Parallel(n_jobs=1)]: Done 3 out of 3 | elapsed: 44.7s remaining: 0.0s
[CV] boosting=gbdt, n_estimators=100, num_leaves=21, score=0.797, total= 7.0s
[CV] boosting=gbdt, n_estimators=100, num_leaves=21 ..................
[Parallel(n_jobs=1)]: Done 4 out of 4 | elapsed: 51.7s remaining: 0.0s
[CV] boosting=gbdt, n_estimators=100, num_leaves=21, score=0.796, total= 6.8s
[CV] boosting=gbdt, n_estimators=100, num_leaves=31 ..................
[Parallel(n_jobs=1)]: Done 5 out of 5 | elapsed: 58.5s remaining: 0.0s
[CV] boosting=gbdt, n_estimators=100, num_leaves=31, score=0.797, total= 7.5s
[CV] boosting=gbdt, n_estimators=100, num_leaves=31 ..................
[Parallel(n_jobs=1)]: Done 6 out of 6 | elapsed: 1.1min remaining: 0.0s
[CV] boosting=gbdt, n_estimators=100, num_leaves=31, score=0.797, total= 7.5s
[CV] boosting=gbdt, n_estimators=100, num_leaves=31 ..................
[Parallel(n_jobs=1)]: Done 7 out of 7 | elapsed: 1.2min remaining: 0.0s
[CV] boosting=gbdt, n_estimators=100, num_leaves=31, score=0.797, total= 7.5s
[CV] boosting=gbdt, n_estimators=100, num_leaves=31 ..................
[Parallel(n_jobs=1)]: Done 8 out of 8 | elapsed: 1.4min remaining: 0.0s
[CV] boosting=gbdt, n_estimators=100, num_leaves=31, score=0.797, total= 7.6s
[CV] boosting=gbdt, n_estimators=100, num_leaves=31 ..................
[Parallel(n_jobs=1)]: Done 9 out of 9 | elapsed: 1.5min remaining: 0.0s
[CV] boosting=gbdt, n_estimators=100, num_leaves=31, score=0.797, total= 7.6s
[CV] boosting=gbdt, n_estimators=100, num_leaves=41 ..................
[CV] boosting=gbdt, n_estimators=100, num_leaves=41, score=0.797, total= 8.0s
[CV] boosting=gbdt, n_estimators=100, num_leaves=41 ..................
[CV] boosting=gbdt, n_estimators=100, num_leaves=41, score=0.797, total= 8.7s
[CV] boosting=gbdt, n_estimators=100, num_leaves=41 ..................
[CV] boosting=gbdt, n_estimators=100, num_leaves=41, score=0.797, total= 8.2s
[CV] boosting=gbdt, n_estimators=100, num_leaves=41 ..................
[CV] boosting=gbdt, n_estimators=100, num_leaves=41, score=0.797, total= 8.1s
[CV] boosting=gbdt, n_estimators=100, num_leaves=41 ..................
[CV] boosting=gbdt, n_estimators=100, num_leaves=41, score=0.797, total= 8.3s
[CV] boosting=gbdt, n_estimators=200, num_leaves=21 ..................
[CV] boosting=gbdt, n_estimators=200, num_leaves=21, score=0.798, total= 10.5s
[CV] boosting=gbdt, n_estimators=200, num_leaves=21 ..................
[CV] boosting=gbdt, n_estimators=200, num_leaves=21, score=0.797, total= 11.5s
[CV] boosting=gbdt, n_estimators=200, num_leaves=21 ..................
[CV] boosting=gbdt, n_estimators=200, num_leaves=21, score=0.797, total= 10.0s
[CV] boosting=gbdt, n_estimators=200, num_leaves=21 ..................
[CV] boosting=gbdt, n_estimators=200, num_leaves=21, score=0.797, total= 10.1s
[CV] boosting=gbdt, n_estimators=200, num_leaves=21 ..................
[CV] boosting=gbdt, n_estimators=200, num_leaves=21, score=0.797, total= 10.1s
[CV] boosting=gbdt, n_estimators=200, num_leaves=31 ..................
[CV] boosting=gbdt, n_estimators=200, num_leaves=31, score=0.798, total= 10.7s
[CV] boosting=gbdt, n_estimators=200, num_leaves=31 ..................
[CV] boosting=gbdt, n_estimators=200, num_leaves=31, score=0.797, total= 10.6s
[CV] boosting=gbdt, n_estimators=200, num_leaves=31 ..................
[CV] boosting=gbdt, n_estimators=200, num_leaves=31, score=0.798, total= 10.8s
[CV] boosting=gbdt, n_estimators=200, num_leaves=31 ..................
[CV] boosting=gbdt, n_estimators=200, num_leaves=31, score=0.797, total= 10.7s
[CV] boosting=gbdt, n_estimators=200, num_leaves=31 ..................
[CV] boosting=gbdt, n_estimators=200, num_leaves=31, score=0.797, total= 10.8s
[CV] boosting=gbdt, n_estimators=200, num_leaves=41 ..................
[CV] boosting=gbdt, n_estimators=200, num_leaves=41, score=0.798, total= 11.4s
[CV] boosting=gbdt, n_estimators=200, num_leaves=41 ..................
[CV] boosting=gbdt, n_estimators=200, num_leaves=41, score=0.797, total= 11.4s
[CV] boosting=gbdt, n_estimators=200, num_leaves=41 ..................
[CV] boosting=gbdt, n_estimators=200, num_leaves=41, score=0.798, total= 11.2s
[CV] boosting=gbdt, n_estimators=200, num_leaves=41 ..................
[CV] boosting=gbdt, n_estimators=200, num_leaves=41, score=0.797, total= 11.2s
[CV] boosting=gbdt, n_estimators=200, num_leaves=41 ..................
[CV] boosting=gbdt, n_estimators=200, num_leaves=41, score=0.797, total= 11.5s
[CV] boosting=gbdt, n_estimators=300, num_leaves=21 ..................
[CV] boosting=gbdt, n_estimators=300, num_leaves=21, score=0.798, total= 12.5s
[CV] boosting=gbdt, n_estimators=300, num_leaves=21 ..................
[CV] boosting=gbdt, n_estimators=300, num_leaves=21, score=0.797, total= 12.6s
[CV] boosting=gbdt, n_estimators=300, num_leaves=21 ..................
[CV] boosting=gbdt, n_estimators=300, num_leaves=21, score=0.798, total= 12.4s
[CV] boosting=gbdt, n_estimators=300, num_leaves=21 ..................
[CV] boosting=gbdt, n_estimators=300, num_leaves=21, score=0.798, total= 12.5s
[CV] boosting=gbdt, n_estimators=300, num_leaves=21 ..................
[CV] boosting=gbdt, n_estimators=300, num_leaves=21, score=0.797, total= 12.5s
[CV] boosting=gbdt, n_estimators=300, num_leaves=31 ..................
[CV] boosting=gbdt, n_estimators=300, num_leaves=31, score=0.798, total= 14.0s
[CV] boosting=gbdt, n_estimators=300, num_leaves=31 ..................
[CV] boosting=gbdt, n_estimators=300, num_leaves=31, score=0.797, total= 13.4s
[CV] boosting=gbdt, n_estimators=300, num_leaves=31 ..................
[CV] boosting=gbdt, n_estimators=300, num_leaves=31, score=0.798, total= 13.1s
[CV] boosting=gbdt, n_estimators=300, num_leaves=31 ..................
[CV] boosting=gbdt, n_estimators=300, num_leaves=31, score=0.797, total= 14.1s
[CV] boosting=gbdt, n_estimators=300, num_leaves=31 ..................
[CV] boosting=gbdt, n_estimators=300, num_leaves=31, score=0.797, total= 13.7s
[CV] boosting=gbdt, n_estimators=300, num_leaves=41 ..................
[CV] boosting=gbdt, n_estimators=300, num_leaves=41, score=0.798, total= 14.6s
[CV] boosting=gbdt, n_estimators=300, num_leaves=41 ..................
[CV] boosting=gbdt, n_estimators=300, num_leaves=41, score=0.797, total= 14.7s
[CV] boosting=gbdt, n_estimators=300, num_leaves=41 ..................
[CV] boosting=gbdt, n_estimators=300, num_leaves=41, score=0.798, total= 14.8s
[CV] boosting=gbdt, n_estimators=300, num_leaves=41 ..................
[CV] boosting=gbdt, n_estimators=300, num_leaves=41, score=0.797, total= 14.3s
[CV] boosting=gbdt, n_estimators=300, num_leaves=41 ..................
[CV] boosting=gbdt, n_estimators=300, num_leaves=41, score=0.797, total= 13.9s
[CV] boosting=dart, n_estimators=100, num_leaves=21 ..................
[CV] boosting=dart, n_estimators=100, num_leaves=21, score=0.795, total= 10.2s
[CV] boosting=dart, n_estimators=100, num_leaves=21 ..................
[CV] boosting=dart, n_estimators=100, num_leaves=21, score=0.795, total= 10.1s
[CV] boosting=dart, n_estimators=100, num_leaves=21 ..................
[CV] boosting=dart, n_estimators=100, num_leaves=21, score=0.795, total= 10.0s
[CV] boosting=dart, n_estimators=100, num_leaves=21 ..................
[CV] boosting=dart, n_estimators=100, num_leaves=21, score=0.795, total= 10.0s
[CV] boosting=dart, n_estimators=100, num_leaves=21 ..................
[CV] boosting=dart, n_estimators=100, num_leaves=21, score=0.795, total= 10.2s
[CV] boosting=dart, n_estimators=100, num_leaves=31 ..................
[CV] boosting=dart, n_estimators=100, num_leaves=31, score=0.795, total= 10.7s
[CV] boosting=dart, n_estimators=100, num_leaves=31 ..................
[CV] boosting=dart, n_estimators=100, num_leaves=31, score=0.795, total= 11.2s
[CV] boosting=dart, n_estimators=100, num_leaves=31 ..................
[CV] boosting=dart, n_estimators=100, num_leaves=31, score=0.796, total= 11.9s
[CV] boosting=dart, n_estimators=100, num_leaves=31 ..................
[CV] boosting=dart, n_estimators=100, num_leaves=31, score=0.795, total= 11.4s
[CV] boosting=dart, n_estimators=100, num_leaves=31 ..................
[CV] boosting=dart, n_estimators=100, num_leaves=31, score=0.796, total= 11.6s
[CV] boosting=dart, n_estimators=100, num_leaves=41 ..................
[CV] boosting=dart, n_estimators=100, num_leaves=41, score=0.796, total= 12.3s
[CV] boosting=dart, n_estimators=100, num_leaves=41 ..................
[CV] boosting=dart, n_estimators=100, num_leaves=41, score=0.795, total= 12.7s
[CV] boosting=dart, n_estimators=100, num_leaves=41 ..................
[CV] boosting=dart, n_estimators=100, num_leaves=41, score=0.796, total= 13.6s
[CV] boosting=dart, n_estimators=100, num_leaves=41 ..................
[CV] boosting=dart, n_estimators=100, num_leaves=41, score=0.795, total= 12.4s
[CV] boosting=dart, n_estimators=100, num_leaves=41 ..................
[CV] boosting=dart, n_estimators=100, num_leaves=41, score=0.795, total= 11.8s
[CV] boosting=dart, n_estimators=200, num_leaves=21 ..................
[CV] boosting=dart, n_estimators=200, num_leaves=21, score=0.796, total= 23.9s
[CV] boosting=dart, n_estimators=200, num_leaves=21 ..................
[CV] boosting=dart, n_estimators=200, num_leaves=21, score=0.795, total= 23.6s
[CV] boosting=dart, n_estimators=200, num_leaves=21 ..................
[CV] boosting=dart, n_estimators=200, num_leaves=21, score=0.796, total= 24.8s
[CV] boosting=dart, n_estimators=200, num_leaves=21 ..................
[CV] boosting=dart, n_estimators=200, num_leaves=21, score=0.795, total= 24.8s
[CV] boosting=dart, n_estimators=200, num_leaves=21 ..................
[CV] boosting=dart, n_estimators=200, num_leaves=21, score=0.796, total= 24.4s
[CV] boosting=dart, n_estimators=200, num_leaves=31 ..................
[CV] boosting=dart, n_estimators=200, num_leaves=31, score=0.796, total= 27.8s
[CV] boosting=dart, n_estimators=200, num_leaves=31 ..................
[CV] boosting=dart, n_estimators=200, num_leaves=31, score=0.796, total= 28.9s
[CV] boosting=dart, n_estimators=200, num_leaves=31 ..................
[CV] boosting=dart, n_estimators=200, num_leaves=31, score=0.796, total= 27.6s
[CV] boosting=dart, n_estimators=200, num_leaves=31 ..................
[CV] boosting=dart, n_estimators=200, num_leaves=31, score=0.796, total= 28.3s
[CV] boosting=dart, n_estimators=200, num_leaves=31 ..................
[CV] boosting=dart, n_estimators=200, num_leaves=31, score=0.796, total= 28.7s
[CV] boosting=dart, n_estimators=200, num_leaves=41 ..................
[CV] boosting=dart, n_estimators=200, num_leaves=41, score=0.797, total= 30.6s
[CV] boosting=dart, n_estimators=200, num_leaves=41 ..................
[CV] boosting=dart, n_estimators=200, num_leaves=41, score=0.796, total= 31.4s
[CV] boosting=dart, n_estimators=200, num_leaves=41 ..................
[CV] boosting=dart, n_estimators=200, num_leaves=41, score=0.796, total= 31.4s
[CV] boosting=dart, n_estimators=200, num_leaves=41 ..................
[CV] boosting=dart, n_estimators=200, num_leaves=41, score=0.796, total= 30.2s
[CV] boosting=dart, n_estimators=200, num_leaves=41 ..................
[CV] boosting=dart, n_estimators=200, num_leaves=41, score=0.796, total= 30.6s
[CV] boosting=dart, n_estimators=300, num_leaves=21 ..................
[CV] boosting=dart, n_estimators=300, num_leaves=21, score=0.796, total= 43.5s
[CV] boosting=dart, n_estimators=300, num_leaves=21 ..................
[CV] boosting=dart, n_estimators=300, num_leaves=21, score=0.796, total= 41.9s
[CV] boosting=dart, n_estimators=300, num_leaves=21 ..................
[CV] boosting=dart, n_estimators=300, num_leaves=21, score=0.796, total= 42.6s
[CV] boosting=dart, n_estimators=300, num_leaves=21 ..................
[CV] boosting=dart, n_estimators=300, num_leaves=21, score=0.796, total= 42.9s
[CV] boosting=dart, n_estimators=300, num_leaves=21 ..................
[CV] boosting=dart, n_estimators=300, num_leaves=21, score=0.796, total= 42.9s
[CV] boosting=dart, n_estimators=300, num_leaves=31 ..................
[CV] boosting=dart, n_estimators=300, num_leaves=31, score=0.797, total= 48.3s
[CV] boosting=dart, n_estimators=300, num_leaves=31 ..................
[CV] boosting=dart, n_estimators=300, num_leaves=31, score=0.796, total= 47.8s
[CV] boosting=dart, n_estimators=300, num_leaves=31 ..................
[CV] boosting=dart, n_estimators=300, num_leaves=31, score=0.797, total= 47.9s
[CV] boosting=dart, n_estimators=300, num_leaves=31 ..................
[CV] boosting=dart, n_estimators=300, num_leaves=31, score=0.797, total= 50.9s
[CV] boosting=dart, n_estimators=300, num_leaves=31 ..................
[CV] boosting=dart, n_estimators=300, num_leaves=31, score=0.797, total= 50.3s
[CV] boosting=dart, n_estimators=300, num_leaves=41 ..................
[CV] boosting=dart, n_estimators=300, num_leaves=41, score=0.797, total= 52.9s
[CV] boosting=dart, n_estimators=300, num_leaves=41 ..................
[CV] boosting=dart, n_estimators=300, num_leaves=41, score=0.797, total= 53.5s
[CV] boosting=dart, n_estimators=300, num_leaves=41 ..................
[CV] boosting=dart, n_estimators=300, num_leaves=41, score=0.797, total= 54.6s
[CV] boosting=dart, n_estimators=300, num_leaves=41 ..................
[CV] boosting=dart, n_estimators=300, num_leaves=41, score=0.797, total= 54.6s
[CV] boosting=dart, n_estimators=300, num_leaves=41 ..................
1
2
#pickle.dump(lgb_score_tuned,open('../models/lgb_score_tuned.pkl','wb'))
lgb_score_tuned=pickle.load(open('../models/lgb_score_tuned.pkl','rb'))
1
plot_roc_curve(lgb_score_tuned, x_test,y_test)
1
<sklearn.metrics._plot.roc_curve.RocCurveDisplay at 0x1fd39ac4dd8>
1
lgb_score.best_estimator_
1
2
3
4
5
6
LGBMClassifier(boosting_type='gbdt', class_weight=None, colsample_bytree=1.0,
importance_type='split', learning_rate=0.1, max_depth=-1,
min_child_samples=20, min_child_weight=0.001, min_split_gain=0.0,
n_estimators=200, n_jobs=4, num_leaves=31, objective='binary',
random_state=None, reg_alpha=0.0, reg_lambda=0.0, silent=False,
subsample=1.0, subsample_for_bin=200000, subsample_freq=0)
1
target_names = ['Good', 'Default']
1
2
3
print(classification_report(y_test, lr_gridSearch.predict(x_test), target_names=target_names))
print(classification_report(y_test, lgb_score.predict(x_test), target_names=target_names))
print(classification_report(y_test, lgb_score_tuned.predict(x_test), target_names=target_names))
1
2
3
4
5
6
7
8
9
10
11
12
13
14
15
16
17
18
19
20
21
22
23
24
25
26
precision recall f1-score support
Good 0.81 0.97 0.88 132915
Default 0.55 0.12 0.19 35317
accuracy 0.79 168232
macro avg 0.68 0.55 0.54 168232
weighted avg 0.75 0.79 0.74 168232
precision recall f1-score support
Good 0.81 0.98 0.88 132915
Default 0.58 0.12 0.20 35317
accuracy 0.80 168232
macro avg 0.70 0.55 0.54 168232
weighted avg 0.76 0.80 0.74 168232
precision recall f1-score support
Good 0.81 0.98 0.88 132915
Default 0.58 0.13 0.21 35317
accuracy 0.80 168232
macro avg 0.69 0.55 0.54 168232
weighted avg 0.76 0.80 0.74 168232
Since precision/recall of default quite low, need to try other option
- Try some feature engineering
- Try with balanced data set
1
cols_to_use = [x for x in df_all_process.columns if 'addr_state_' not in x]
1
len(cols_to_use)
1
67
1
2
3
x_train, x_test,y_train,y_test = train_test_split(df_all_process[cols_to_use].drop('loan_status', axis=1),\
df_all_process.loan_status,test_size=.2,\
shuffle=True,stratify=df_all_process.loan_status,random_state=20)
1
2
3
4
5
6
7
8
9
def create_resample(X,y):
return_dict= {}
ros=RandomOverSampler(random_state=20)
return_dict['train_ros'], return_dict['y_ros'] = ros.fit_resample(X, y)
rus=RandomUnderSampler(random_state=20)
return_dict['train_rus'], return_dict['y_rus'] = rus.fit_resample(X,y)
smote=SMOTE(random_state=20)
return_dict['train_smote'], return_dict['y_smote'] = smote.fit_resample(X,y)
return return_dict
1
2
%%time
train_dict=create_resample(x_train, y_train)
1
2
3
4
5
6
Function safe_indexing is deprecated; safe_indexing is deprecated in version 0.22 and will be removed in version 0.24.
Function safe_indexing is deprecated; safe_indexing is deprecated in version 0.22 and will be removed in version 0.24.
Function safe_indexing is deprecated; safe_indexing is deprecated in version 0.22 and will be removed in version 0.24.
Wall time: 24min 9s
1
train_dict.keys()
1
dict_keys(['train_ros', 'y_ros', 'train_rus', 'y_rus', 'train_smote', 'y_smote'])
1
train_dict['train_ros']
1
2
3
4
5
6
7
8
9
10
11
12
13
array([[ 0.41244608, -0.56157167, -0.49256411, ..., 0. ,
0. , 0. ],
[-1.10824679, -0.56157167, -0.26365397, ..., 0. ,
0. , 0. ],
[ 0.17849333, -0.56157167, -1.41248368, ..., 0. ,
0. , 0. ],
...,
[ 0.3247138 , 1.78071663, 1.16970898, ..., 0. ,
0. , 0. ],
[-0.28941217, -0.56157167, -0.82416305, ..., 0. ,
0. , 0. ],
[-1.41238537, -0.56157167, 1.07343857, ..., 0. ,
0. , 0. ]])
Random Over Sampling
1
2
3
4
5
6
7
8
9
10
11
%%time
lgb_classifier = lgb.LGBMClassifier(objective='binary', n_jobs=4, silent=False)
lgb_param = [
{
#'num_leaves': [31,7],
'n_estimators': [100,200],
'learning_rate': [.01,.1]
}
]
lgb_ros_score = GridSearchCV(lgb_classifier,lgb_param,cv=5,verbose=10)
1
2
%%time
lgb_ros_score.fit(train_dict['train_ros'],train_dict['y_ros'])
1
2
#pickle.dump(lgb_ros_score,open('../models/lgb_ros_score.pkl','wb'))
lgb_ros_score=pickle.load(open('../models/lgb_ros_score.pkl','rb'))
1
plot_roc_curve(lgb_ros_score, x_test,y_test)
1
<sklearn.metrics._plot.roc_curve.RocCurveDisplay at 0x22f471b97f0>
1
2
target_names = ['Good', 'Default']
print(classification_report(y_test, lgb_ros_score.predict(x_test), target_names=target_names))
1
2
3
4
5
6
7
8
precision recall f1-score support
Good 0.88 0.66 0.75 132915
Default 0.34 0.68 0.46 35317
accuracy 0.66 168232
macro avg 0.61 0.67 0.60 168232
weighted avg 0.77 0.66 0.69 168232
Random under sampling
1
2
3
4
5
6
7
8
9
10
11
%%time
lgb_classifier = lgb.LGBMClassifier(objective='binary', n_jobs=4, silent=False)
lgb_param = [
{
#'num_leaves': [31,7],
'n_estimators': [100,200],
'learning_rate': [.01,.1]
}
]
lgb_rus_score = GridSearchCV(lgb_classifier,lgb_param,cv=5,verbose=10)
1
2
%%time
lgb_rus_score.fit(train_dict['train_rus'],train_dict['y_rus'])
1
2
#pickle.dump(lgb_rus_score,open('../models/lgb_rus_score.pkl','wb'))
lgb_rus_score=pickle.load(open('../models/lgb_rus_score.pkl','rb'))
1
plot_roc_curve(lgb_rus_score, x_test,y_test)
1
<sklearn.metrics._plot.roc_curve.RocCurveDisplay at 0x22f474ba080>
1
print(classification_report(y_test, lgb_rus_score.predict(x_test), target_names=target_names))
1
2
3
4
5
6
7
8
precision recall f1-score support
Good 0.88 0.65 0.75 132915
Default 0.34 0.68 0.45 35317
accuracy 0.66 168232
macro avg 0.61 0.67 0.60 168232
weighted avg 0.77 0.66 0.69 168232
SMOTE Sampling
1
2
3
4
5
6
7
8
9
10
11
%%time
lgb_classifier = lgb.LGBMClassifier(objective='binary', n_jobs=4, silent=False)
lgb_param = [
{
#'num_leaves': [31,7],
'n_estimators': [100,200],
'learning_rate': [.01,.1]
}
]
lgb_smote_score = GridSearchCV(lgb_classifier,lgb_param,cv=5,verbose=10)
1
2
%%time
lgb_smote_score.fit(train_dict['train_smote'],train_dict['y_smote'])
1
2
#pickle.dump(lgb_smote_score,open('../models/lgb_smote_score.pkl','wb'))
lgb_smote_score=pickle.load(open('../models/lgb_smote_score.pkl','rb'))
1
plot_roc_curve(lgb_smote_score, x_test,y_test)
1
<sklearn.metrics._plot.roc_curve.RocCurveDisplay at 0x22f52652da0>
1
print(classification_report(y_test, lgb_smote_score.predict(x_test), target_names=target_names))
1
2
3
4
5
6
7
8
precision recall f1-score support
Good 0.81 0.98 0.88 132915
Default 0.57 0.12 0.19 35317
accuracy 0.80 168232
macro avg 0.69 0.55 0.54 168232
weighted avg 0.76 0.80 0.74 168232
SHAP
1
2
3
4
5
X=pd.DataFrame(train_dict['train_ros'],columns=x_train.columns)
X_y= X.copy()
X_y['y']=train_dict['y_ros']
X_y['y_hat']=model.predict(X)
X_y[(X_y.y==1)&(X_y.y_hat==1)].head()
loan_amnt | term | int_rate | installment | emp_length | annual_inc | dti | delinq_2yrs | fico_range_low | inq_last_6mths | mths_since_last_delinq | open_acc | pub_rec | revol_bal | revol_util | total_acc | mort_acc | num_accts_ever_120_pd | num_actv_bc_tl | num_actv_rev_tl | num_bc_sats | num_bc_tl | num_il_tl | num_op_rev_tl | num_rev_accts | num_rev_tl_bal_gt_0 | num_sats | num_tl_120dpd_2m | num_tl_30dpd | num_tl_90g_dpd_24m | num_tl_op_past_12m | pct_tl_nvr_dlq | percent_bc_gt_75 | pub_rec_bankruptcies | tax_liens | tot_hi_cred_lim | total_bal_ex_mort | total_bc_limit | total_il_high_credit_limit | earliest_cr_line_yrs | num_un_sats | grade | sub_grade | home_ownership_ANY | home_ownership_MORTGAGE | home_ownership_NONE | home_ownership_OTHER | home_ownership_OWN | home_ownership_RENT | verification_status_Not Verified | verification_status_Source Verified | verification_status_Verified | purpose_car | purpose_credit_card | purpose_debt_consolidation | purpose_educational | purpose_home_improvement | purpose_house | purpose_major_purchase | purpose_medical | purpose_moving | purpose_other | purpose_renewable_energy | purpose_small_business | purpose_vacation | purpose_wedding | y | y_hat | |
---|---|---|---|---|---|---|---|---|---|---|---|---|---|---|---|---|---|---|---|---|---|---|---|---|---|---|---|---|---|---|---|---|---|---|---|---|---|---|---|---|---|---|---|---|---|---|---|---|---|---|---|---|---|---|---|---|---|---|---|---|---|---|---|---|---|---|---|---|
21 | -1.295409 | -0.561572 | 1.011397 | -1.238437 | 1.127142 | -0.387824 | -0.050499 | -0.362172 | 0.173196 | 2.352020 | 0.992706 | 0.066160 | -0.359792 | -0.430947 | -0.279377 | -0.878959 | -0.358282 | -0.379514 | -0.746492 | 0.114361 | 0.104998 | -0.695487 | -0.629753 | 0.397948 | -0.613865 | 0.130822 | 0.071395 | -0.026606 | -0.054514 | -0.173928 | 1.557939 | 0.663821 | -0.724567 | -0.358433 | -0.126956 | -0.359522 | -0.607690 | -0.692055 | -0.504581 | 0.240152 | -0.170197 | 3.0 | 17.0 | 0.0 | 1.0 | 0.0 | 0.0 | 0.0 | 0.0 | 0.0 | 0.0 | 1.0 | 1.0 | 0.0 | 0.0 | 0.0 | 0.0 | 0.0 | 0.0 | 0.0 | 0.0 | 0.0 | 0.0 | 0.0 | 0.0 | 0.0 | 1 | 1 |
27 | 1.816163 | 1.780717 | 0.031576 | 0.973863 | 1.127142 | 0.296545 | 0.402269 | -0.362172 | 0.173196 | -0.720325 | -1.011293 | 1.370300 | -0.359792 | 0.209428 | 0.362714 | 0.539599 | 0.126903 | -0.379514 | -0.285074 | 0.743729 | -0.246077 | -0.277608 | 1.024236 | 0.170276 | -0.113617 | 0.774460 | 1.387986 | -0.026606 | -0.054514 | -0.173928 | 1.002394 | 0.292437 | 0.110152 | -0.358433 | -0.126956 | 0.227897 | 0.226966 | 0.121670 | 0.513495 | 0.511073 | -0.170197 | 2.0 | 12.0 | 0.0 | 0.0 | 0.0 | 0.0 | 1.0 | 0.0 | 0.0 | 1.0 | 0.0 | 0.0 | 1.0 | 0.0 | 0.0 | 0.0 | 0.0 | 0.0 | 0.0 | 0.0 | 0.0 | 0.0 | 0.0 | 0.0 | 0.0 | 1 | 1 |
29 | 0.412446 | 1.780717 | 0.741839 | 0.019619 | -0.967972 | 0.173788 | 0.798168 | 1.921915 | -0.799511 | -0.720325 | -1.058958 | 1.556605 | -0.359792 | -0.201650 | -0.523786 | -0.044513 | -0.843467 | -0.379514 | 1.560602 | 1.687783 | 0.807146 | 0.140271 | -0.354088 | 1.991651 | 0.511693 | 1.739918 | 1.576071 | -0.026606 | -0.054514 | -0.173928 | 0.446849 | -0.294590 | 0.705585 | -0.358433 | -0.126956 | -0.474600 | -0.133920 | -0.547607 | 0.456689 | 1.188375 | -0.170197 | 3.0 | 17.0 | 0.0 | 0.0 | 0.0 | 0.0 | 0.0 | 1.0 | 0.0 | 1.0 | 0.0 | 0.0 | 0.0 | 1.0 | 0.0 | 0.0 | 0.0 | 0.0 | 0.0 | 0.0 | 0.0 | 0.0 | 0.0 | 0.0 | 0.0 | 1 | 1 |
56 | -0.757318 | -0.561572 | -0.113900 | -0.670700 | 1.127142 | 0.925674 | -0.177362 | -0.362172 | -1.123746 | 0.303790 | 0.992706 | 1.183994 | -0.359792 | 0.442548 | 0.963381 | 0.205820 | -0.843467 | -0.379514 | 2.483440 | 2.002467 | 1.860368 | 0.558150 | -0.216256 | 1.536307 | 0.761817 | 2.061738 | 1.199902 | -0.026606 | -0.054514 | -0.173928 | -0.108696 | 0.663821 | 0.388392 | -0.358433 | -0.126956 | -0.482011 | 0.451116 | 0.299822 | 0.310625 | -0.437149 | -0.170197 | 2.0 | 11.0 | 0.0 | 0.0 | 0.0 | 0.0 | 0.0 | 1.0 | 1.0 | 0.0 | 0.0 | 0.0 | 1.0 | 0.0 | 0.0 | 0.0 | 0.0 | 0.0 | 0.0 | 0.0 | 0.0 | 0.0 | 0.0 | 0.0 | 0.0 | 1 | 1 |
59 | 0.871578 | -0.561572 | -0.755704 | 1.029910 | -1.491750 | -0.117759 | 0.825509 | 1.921915 | 0.011078 | -0.720325 | -1.065175 | 0.625077 | -0.359792 | -0.017593 | -0.072251 | -0.712070 | 0.126903 | -0.379514 | 0.637764 | 0.743729 | 0.807146 | -0.277608 | -0.905418 | 0.625620 | -0.363741 | 0.774460 | 0.635649 | -0.026606 | -0.054514 | -0.173928 | -1.219786 | -0.749835 | -0.485281 | -0.358433 | -0.126956 | 0.088942 | 0.111578 | -0.427233 | 0.121578 | 1.052914 | -0.170197 | 1.0 | 7.0 | 0.0 | 1.0 | 0.0 | 0.0 | 0.0 | 0.0 | 1.0 | 0.0 | 0.0 | 0.0 | 0.0 | 1.0 | 0.0 | 0.0 | 0.0 | 0.0 | 0.0 | 0.0 | 0.0 | 0.0 | 0.0 | 0.0 | 0.0 | 1 | 1 |
1
model=lgb_rus_score.best_estimator_
1
2
%%time
shap_explainer = shap.TreeExplainer(model,X)
1
Wall time: 17.9 s
1
2
%%time
shap_values = shap_explainer.shap_values(X, approximate=True)
1
Wall time: 17.7 s
1
shap.force_plot(shap_explainer.expected_value,shap_values[0,:],X.iloc[0,:])
Visualization omitted, Javascript library not loaded!
Have you run `initjs()` in this notebook? If this notebook was from another user you must also trust this notebook (File -> Trust notebook). If you are viewing this notebook on github the Javascript has been stripped for security. If you are using JupyterLab this error is because a JupyterLab extension has not yet been written.
Have you run `initjs()` in this notebook? If this notebook was from another user you must also trust this notebook (File -> Trust notebook). If you are viewing this notebook on github the Javascript has been stripped for security. If you are using JupyterLab this error is because a JupyterLab extension has not yet been written.
1
shap.force_plot(shap_explainer.expected_value,shap_values[21,:],X.iloc[21,:])
Visualization omitted, Javascript library not loaded!
Have you run `initjs()` in this notebook? If this notebook was from another user you must also trust this notebook (File -> Trust notebook). If you are viewing this notebook on github the Javascript has been stripped for security. If you are using JupyterLab this error is because a JupyterLab extension has not yet been written.
Have you run `initjs()` in this notebook? If this notebook was from another user you must also trust this notebook (File -> Trust notebook). If you are viewing this notebook on github the Javascript has been stripped for security. If you are using JupyterLab this error is because a JupyterLab extension has not yet been written.
1
shap.summary_plot(shap_values, X)
1
1